DeepFakes Impact on SaaS: Insights for Cybersecurity Professionals
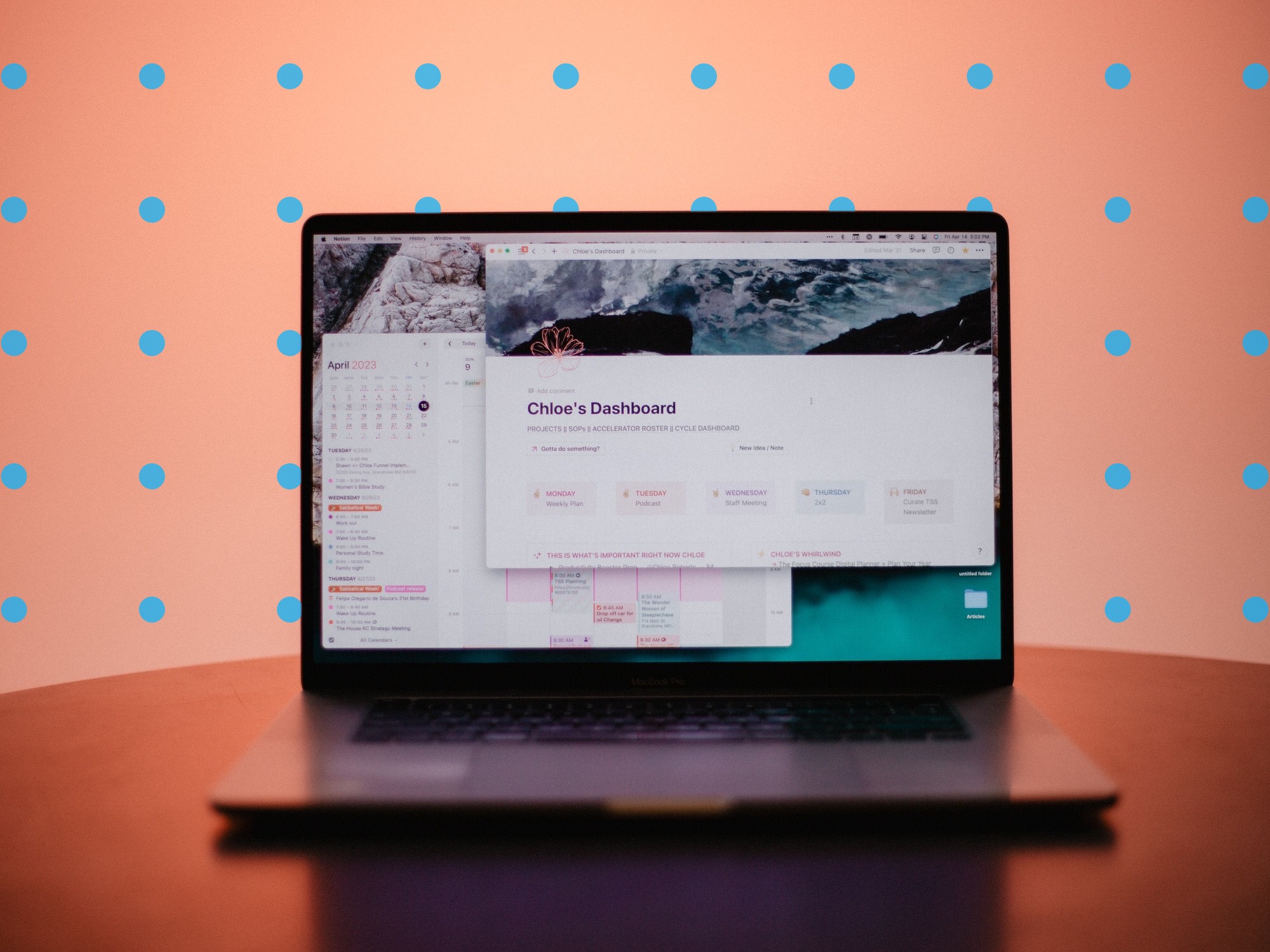
DeepFakes, a rapidly evolving technology that leverages artificial intelligence to create hyper-realistic digital forgeries, poses significant challenges to the SaaS industry. By manipulating images, videos, and audio, these fakes can cause immense damage through disinformation, brand reputation harm, and social engineering. Cybersecurity professionals are increasingly tasked with safeguarding SaaS applications against this mounting threat.
This article specifically targets cybersecurity professionals within the SaaS industry; SaaS CEOs, founders, product managers, technical and product development teams, as well as digital marketers and growth strategists. Each of these stakeholders plays a vital role in maintaining the integrity, security, and reliability of SaaS platforms and the data they process. Understanding how DeepFakes can affect user verification, detection and prevention efforts, and the overall security landscape is paramount.
Throughout the article, we will delve deeper into the intricacies of DeepFakes technology, its implications on cybersecurity, and the challenges associated with detecting and preventing such fraud. By gaining insights into the cutting-edge methods employed by malicious actors, you will be better equipped to stay ahead of this potent threat, ensure secure user interactions, and maintain user trust in your SaaS applications.
As a cybersecurity professional in the SaaS industry, you are instrumental in safeguarding not only your company's digital assets but also the security and privacy of your end-users. Stay tuned for the subsequent sections on understanding DeepFakes technology, mapping the threat landscape, examining DeepFakes-driven fraud techniques and detection challenges, assessing the impact of DeepFakes fraud on cybersecurity goals, and finally exploring effective strategies for detecting and preventing DeepFakes fraud.
Understanding DeepFakes Technology
How DeepFakes are Created
DeepFakes rely on a class of artificial intelligence algorithms known as Generative Adversarial Networks (GANs). GANs consist of two neural networks: a generator and a discriminator. The generator creates fake images or videos by learning to mimic specific datasets, while the discriminator evaluates the generated output to determine if it is real or fake. Through this continuous feedback loop, the generator improves its ability to create increasingly realistic deepfakes.
Large data sets are crucial for training GANs to produce convincing deepfakes. The more images, videos, or audio clips fed into the algorithm, the better it becomes at replicating real-life content. This growth has been accelerated with the increased availability of high-quality data and advancements in machine learning techniques, making it easier for malicious actors to create and distribute deceptive content.
Mapping the Threat Landscape
DeepFakes pose significant challenges as they can generate highly convincing images, videos, and audio content capable of fooling humans and even some automated detection systems. As a result, a wide range of potential harms can arise, including disinformation, reputational damage, and social engineering attacks.
Disinformation can lead to public mistrust, political polarization, and even violence. Reputational harm might occur when a deepfake presents a SaaS CEO or founder engaging in unethical behavior. This scenario can lead to a loss of consumer trust, diminished market share, and other negative effects. Social engineering attacks can exploit deepfakes to manipulate employees into revealing sensitive information or performing unauthorized actions on behalf of the attackers.
The ever-evolving threat landscape brought about by deepfakes requires cybersecurity professionals in the SaaS industry to stay informed and prepared for these emerging challenges to ensure a secure and trustworthy online environment.
DeepFakes-driven Fraud Techniques and Detection Challenges
Tactics Employed by Bad Actors
Bad actors have a wide array of tactics at their disposal, which can be employed to bypass online security measures and drive fraud:
- GANs for realistic image synthesis to create fake-profile pictures
- Manipulating video and audio for impersonation or misinformation campaigns
- Masking IP addresses and device information to evade geographical restrictions
- AI-driven chatbots capable of conducting social engineering attacks
- Automation tools for mass-scale attacks on SaaS platforms
- Evasion techniques to avoid detection by security systems
Why Detection and Prevention are Difficult
Detecting and preventing deepfake-driven fraud is a complex challenge due to the rapid advancements in AI-driven technologies and the increasing sophistication of evasion methods employed by bad actors. Furthermore, the adaptive nature of these attacks makes it difficult for security measures to remain effective over time.
The sheer volume of data and user interactions on SaaS platforms can also make detection even more challenging, as it is harder to identify small-scale fraudulent activity amidst substantial legitimate traffic. Finally, the social nature of many SaaS platforms enables bad actors to leverage social connections to establish trust with potential victims, making it more challenging to identify and prevent fraudulent interactions.
DeepFakes-driven Fraud Techniques and Detection Challenges
Tactics Employed by Bad Actors
DeepFakes technology has opened doors for bad actors to engage in a variety of fraudulent tactics that pose threats to the cybersecurity landscape. These methods include:
- Employing GANs for ultra-realistic image synthesis, particularly for identity theft and impersonation purposes
- Manipulating video and audio recordings to present falsified or out-of-context information, compromising the integrity of user-generated content on SaaS platforms
- Hiding behind masked IP addresses and falsified device information to avoid location-specific restrictions and evade detection
- Utilizing AI-driven chatbots for effective social engineering schemes, tricking users into providing sensitive information or access credentials
- Automation for mass-scale attacks, generating and distributing DeepFakes with minimal human intervention
- Employing evasion techniques designed to bypass traditional cybersecurity measures and avoid detection by signature-based security systems
Why Detection and Prevention are Difficult
Detecting and preventing DeepFakes-driven fraud have become increasingly challenging due to several factors:
- Rapid advancements in AI-driven technologies are enhancing the quality and realism of DeepFakes, making it harder for humans and traditional security systems to differentiate between fake and genuine content
- The sophistication and variety of evasion methods continue to advance, outpacing traditional cybersecurity measures
- Attacks leveraging DeepFakes are adaptive in nature, often utilizing trial-and-error to identify vulnerabilities and potential weaknesses in cybersecurity systems
- The volume of data and user interactions on SaaS platforms continues to grow exponentially, creating multiple entry points and opportunities for malicious activities by bad actors
The evolving complexity of DeepFakes-driven fraud techniques and detection challenges highlight the need for advanced user verification technologies and adaptive cybersecurity measures tailored to the unique threats posed by DeepFakes in the SaaS industry.
Get started with Verisoul for free
Compromised User Verification Processes
DeepFakes-driven fraud can have multiple effects on SaaS, one of which is compromising user verification processes. Traditional user verification methods, such as email confirmation, two-factor authentication, and knowledge-based authentication, may no longer be sufficient to protect businesses from fake users. DeepFakes' ability to generate realistic images, videos, and audio files can be used to bypass these methods, leading to unauthorized access to SaaS platforms and sensitive data manipulation.
- Bypassing email confirmation: Fraudsters may use AI-generated profiles to create credible email accounts, which can pass standard email verification checks.
- Overcoming two-factor authentication: DeepFakes can assist in impersonating genuine users, making it possible for bad actors to intercept or spoof authentication codes.
- Defeating knowledge-based authentication: Machine learning algorithms can scrape the internet for personal information, allowing fraudsters to answer security questions accurately.
Hindered Detection and Erosion of User Trust
Continuous evolution and improvement of DeepFakes technology make it increasingly difficult to identify fake images, videos, or audio files. The inability to detect DeepFakes can lead to a decline in user trust, as they question the authenticity of online interactions and content. This erosion of trust can damage brand reputation, create customer churn, and discourage new users from engaging with SaaS platforms.
- Hesitation to trust online content: Users may be wary of engaging with businesses that cannot guarantee the authenticity of their platform's content, leading to a decline in customer loyalty and retention.
- Decreased brand reputation: SaaS companies that fail to address DeepFakes-driven fraud may be perceived as negligent or insecure, impacting their credibility and authority in their industry.
Compliance Risks and Legal Implications
The presence of DeepFakes-driven fraud introduces new legal challenges and compliance risks for SaaS companies. They need to ensure they are adhering to data protection laws and industry-specific regulations while combating fraud driven by DeepFakes. Failure to address these threats can result in legal and financial ramifications, such as fines, penalties, and reputational harm.
- Data protection laws: The General Data Protection Regulation (GDPR) and California Consumer Privacy Act (CCPA), among other data protection laws, require businesses to protect user data from unauthorized access or misuse. DeepFakes-driven fraud can violate these regulations by manipulating sensitive user information or facilitating unauthorized access.
- Industry-specific regulations: SaaS companies operating within regulated industries (e.g., healthcare, finance) have even more stringent guidelines for securing user data and maintaining privacy. Failure to protect against DeepFakes-driven fraud jeopardizes their ability to stay compliant with these regulations.
- Legal consequences: Companies that fail to address DeepFakes fraud may face legal action from affected users or regulatory entities, leading to financial penalties and reputational damage.
Clearly, the implications of DeepFakes-driven fraud on cybersecurity goals and challenges are far-reaching. SaaS companies must prioritize advanced user verification processes and adapt to the changing technological landscape to protect their platforms, data, and user trust.
Effective Strategies for Detecting and Preventing DeepFakes Fraud
Despite the challenges posed by DeepFakes in the SaaS industry, there are effective strategies that can help in detecting and preventing DeepFakes fraud. Combining advanced user verification techniques and adapting to the evolving threat landscape will ensure greater security for businesses and users.
Advanced User Verification Techniques
To counter the growing sophistication and realism of DeepFakes-generated images and videos, measures should be taken to strengthen user verification processes. Some advanced techniques include:
-
Real-time, multi-factor authentication: Using a combination of factors like passwords, security tokens, and one-time codes helps prevent unauthorized access. Implementing real-time checks during authentication adds another layer of security.
-
Biometric-based verifications: Utilizing biometric identifiers like fingerprints, facial recognition, and voice analysis for authentication can make it difficult for DeepFakes to bypass these verification methods.
-
Liveness detection: Verifying the user's physical presence in real-time, rather than relying on static images or pre-recorded videos, can help in identifying and thwarting DeepFakes. These techniques may include analyzing eye movements, checking for image distortions, or detecting facial expressions.
Adaptation and Integration
As DeepFakes technology advances, so too must the security measures used to detect and prevent fraud. To stay ahead of these evolving threats, businesses must:
-
Monitor and update algorithms: Continuously monitor advancements in the DeepFakes landscape and adapt your cybersecurity measures accordingly. Update detection algorithms and verification methods to ensure they can effectively identify and combat DeepFakes.
-
Seamless integration with existing cybersecurity infrastructure and processes: When adopting new techniques and technologies to combat DeepFakes, ensure that they can be seamlessly integrated into your existing cybersecurity systems. This will help avoid any security gaps and ensure a cohesive approach to eliminating DeepFakes fraud.
In conclusion, the rising prevalence of DeepFakes and their potential impact on the SaaS industry should not be underestimated. By implementing advanced user verification techniques and continuously adapting to the evolving threat landscape, cybersecurity professionals can ensure the integrity and security of SaaS platforms while maintaining user trust.
Final Thoughts and Next Steps
As DeepFakes technology continues to evolve and become more accessible, its potential impact on SaaS platforms must not be underestimated. Now more than ever, it is crucial for cybersecurity professionals to stay informed about the latest developments in the DeepFakes landscape and be prepared to tackle emerging threats.
To effectively combat DeepFakes-driven fraud, the following steps should be prioritized:
-
Explore advanced user verification technologies: Real-time, multi-factor authentication, biometric-based verifications, and liveness detection techniques can provide added layers of security, minimizing the risk of fake users infiltrating your SaaS platform.
-
Continuously evaluate and update security measures: As DeepFakes technology advances, so too must your cybersecurity strategies. Monitor the latest trends and developments, and ensure that your security infrastructure is continuously updated to stay ahead of potential threats.
-
Adaptation and Seamless Integration: Work to integrate advanced verification solutions into your cybersecurity infrastructure and processes without disrupting genuine users' experience. This will help maintain platform security without compromising user trust and satisfaction.
DeepFakes pose a significant and growing challenge to the SaaS industry, but by implementing robust user verification processes and leveraging advanced detection techniques, cybersecurity professionals can defend against the increasing sophistication of DeepFake-driven fraud. The key to keeping your SaaS platform secure is being aware of the risks, investing in the right technologies, and staying vigilant in the face of ever-evolving threats.