DeepFakes Impact on Digital Marketing: Advertisers, Brands, and AI Enthusiasts Beware
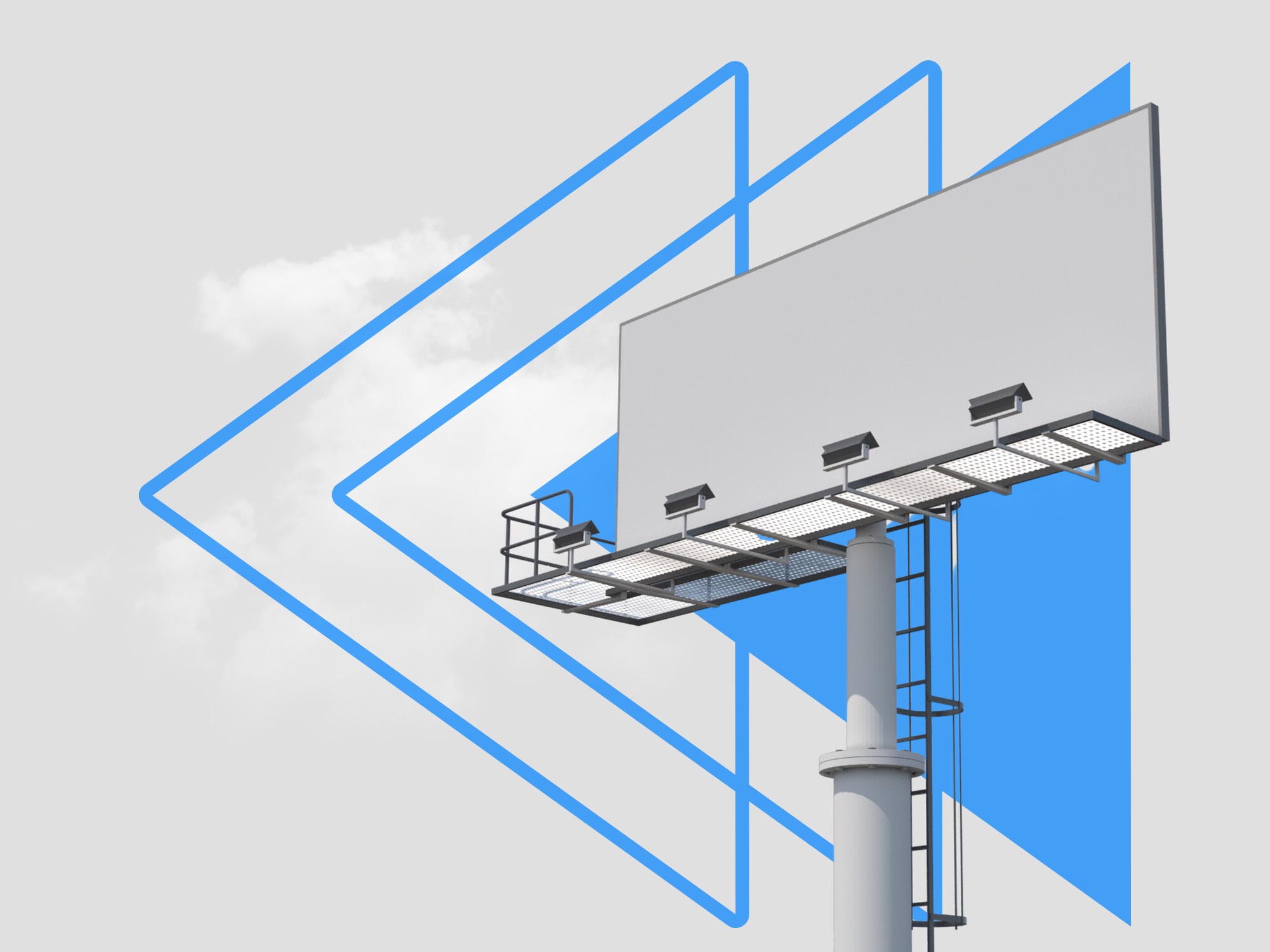
The pervasive rise of deepfake technology has raised concerns in various industries, specifically in the realm of advertising and marketing. These sophisticated AI-generated imitations of real people and events have the potential to impact brand reputation and the effectiveness of marketing campaigns. This article will provide an overview of the top five technical tactics professionals in the advertising landscape can employ to mitigate the risks associated with deepfakes.
Deepfake technology leverages artificial intelligence and deep learning to create, alter, or manipulate visual content, such as images, videos, and audio recordings. This allows the creation of realistic-looking media that can be difficult to differentiate from authentic material. While innovative and intriguing, deepfake poses various ethical concerns and challenges advertisers, content creators, and brands to reevaluate their strategies to combat the disruptive effects of these digital manipulations.
Brand integrity hinges on the ability to maintain consumers' trust. With the pervasiveness of deepfakes, the integrity of the information disseminated through digital channels is put into question, creating an atmosphere of doubt surrounding the authenticity of marketing materials. Advertisers and brands need to understand the implications of deepfake technology on their brand image and devise tactics to eliminate or minimize the risks it presents.
Moreover, digital marketing campaigns necessitate a comprehensive understanding of technologies used to create, distribute, and analyze diverse multimedia content. It is essential for professionals in the field to be well-versed in the intricacies of deepfake and other AI-driven manipulations. Familiarity with robust techniques to detect and address such digital manipulations will contribute to more secure and efficient marketing strategies and ensure consumers are protected from malicious campaigns.
In this article, we will delve into the top five technical tactics to address deepfake threats to advertising and marketing campaigns. These tactics will offer practical insights to digital marketing practitioners, advertisers, brands, AI and technology enthusiasts, media and communication professionals, and media literacy and digital ethics advocates. To safeguard brand reputation and maintain customer trust, it is vital for professionals across these segments to stay ahead of the curve and adapt their strategies to navigate the uncertain landscape of deepfake manipulation.
Strategy 1: Device and Browser Fingerprinting
What is Device and Browser Fingerprinting
Device and Browser Fingerprinting is a technique used to identify unique device and browser characteristics, enabling the monitoring of user behavior patterns to detect potential deepfake-generated content. This process helps in pinpointing suspicious activities by distinguishing genuine users from those using deepfake accounts.
How does it work
Fingerprinting works by collecting various device and browser attributes such as screen resolution, user agent, IP address, and installed software versions. Once collected, these attributes are then analyzed to determine if the user behavior patterns are consistent with authentic human engagement or if they indicate the presence of deepfake accounts.
Pros & cons
Pros:
- Efficient detection of unusual activity: Device and Browser Fingerprinting quickly identifies suspect behavior patterns or device usage, providing an early warning system for detecting deepfake content.
- Exposure of deceptive ad placements: By identifying deepfake-generated content, it can help uncover fraudulent ad placements designed to deceive users and manipulate ad metrics.
Cons:
- Privacy concerns: Some users might perceive this technique as intrusive, raising concerns about user privacy and potential data misuse.
- Possible false positives: As the method relies on several factors, it might occasionally generate false positives, incorrectly flagging legitimate users as potential deepfake accounts.
Tactical implementation
To implement Device and Browser Fingerprinting, consider using fingerprinting libraries like FingerprintJS or OWASP Amass. Such libraries streamline the collection of device and browser attributes, making it easier to integrate the fingerprinting process into your marketing analytics platforms.
Once the data is collected, build a system that analyzes the collected attributes, determines user behavior patterns, and flags suspicious activities potentially related to deepfake content. This system can be incorporated into existing marketing analytics tools, allowing you to monitor and mitigate the risks associated with deepfakes at the same time as analyzing other marketing metrics.
By employing Device and Browser Fingerprinting, digital marketing professionals, advertisers, and brands can take proactive steps to shield their advertising campaigns from the potentially harmful impact of deepfake content. This tactic enables those in the field to better identify and respond to emerging risks while ensuring the integrity of their marketing materials and maintaining consumer trust in their brand.
Strategy 2: Facial Biometrics
What is Facial Biometrics
Facial biometrics is a technology that enables the genuineness verification of faces in visual content like images and videos. It plays a crucial role in addressing the issue of manipulated influencer endorsements or thought-leader recommendations in advertising campaigns, which can potentially harm brand reputation and consumer trust. By leveraging facial biometrics, advertisers can ensure the content they create or endorse is legitimate and free from deepfake manipulations.
How does it work
Facial biometrics work by analyzing facial features and patterns in visual content like images and videos. Algorithms detect and recognize the unique characteristics of a person's face and compare them with an authorized facial database, helping determine the authenticity of the content. If the facial features match the authorized database, the content is considered genuine; otherwise, it is flagged as potentially manipulated or deepfake-generated.
Pros & cons
Pros:
-
Verification of influencer and thought-leader identities: Facial biometrics helps advertisers validate the identity of influencers and other public figures in their marketing campaigns, ensuring that they are not using fake or manipulated content to promote their brand.
-
Detection of counterfeit content: Facial biometrics can detect deepfake-generated content used by malicious actors to deceive consumers, helping protect businesses from brand damage and financial losses due to fraudulent advertising campaigns.
Cons:
-
High storage and computing requirements: Implementing facial biometrics may require significant storage capacity and computational power, which can be expensive and challenging to maintain for smaller businesses or organizations.
-
Dependence on high-quality input images: The effectiveness of facial biometrics relies heavily on the quality of input images or videos, as low-quality content can lead to inaccuracies or false positives in identifying deepfake-generated material.
Tactical implementation
To implement facial biometrics in advertising and marketing activities, businesses can integrate facial recognition APIs like Amazon Rekognition, Microsoft Azure Face API, or similar services. These APIs provide a range of facial analysis functions that can identify, analyze, and match faces in visual content to an authorized database.
Creating a comprehensive authorized facial database is crucial for successful facial biometrics implementation. This database should include high-quality images or videos of influencers, thought leaders, and other public figures involved in the marketing campaigns. It must be updated regularly to account for any changes in facial appearance due to aging, weight fluctuations, or cosmetic procedures.
Once the facial recognition APIs are integrated and the authorized facial database is established, marketers can use facial biometrics to verify the authenticity of any visual content featuring influencers or other endorsers. If the content is flagged as suspicious or deepfake-generated, advertisers can take appropriate action to prevent further damage to their brand reputation and consumer trust.
Get started with Verisoul for free
Strategy 3: 3D Liveness Detection
What is 3D Liveness Detection?
3D Liveness Detection is a validation technique that examines the three-dimensional aspects of video content to ensure its authenticity and prevent deepfake manipulations from infiltrating marketing campaigns. By assessing the depth and motion in video content, this advanced method can identify whether the subjects presented in the video are real humans or fabricated deepfake creations.
How does it work?
3D Liveness Detection analyzes video content to extract depth and motion information, which can be used to identify manipulation attempts or inconsistencies within the media. This information is then compared against established standards to determine whether the video content is authentic or altered. By detecting fabricated deepfake content, advertisers can safeguard their marketing campaigns and retain the trust and credibility of their target audience.
Pros & cons
-
Pros:
- Confirmation of real humans in videos: With 3D Liveness Detection, advertisers can be sure that the individuals depicted in their marketing content are genuine and not created through deepfake technology.
- Protection from manipulated video content: By identifying and blocking deepfake videos, this technique allows brands to maintain the truthfulness and integrity of their advertisements.
-
Cons:
- Applicable to video content only: 3D Liveness Detection can't protect against deepfakes in other forms of media, such as images or audio files.
- Limited accuracy in low-quality videos: This technology might struggle to identify inconsistencies in videos with low resolution or poor lighting conditions, potentially decreasing its effectiveness in detecting deepfake content.
Tactical implementation
To implement 3D Liveness Detection in marketing efforts, businesses should consider the following steps:
-
Integrate 3D liveness detection SDKs: Leverage technology provided by companies like FaceTec or ID R&D to incorporate their liveness detection solutions into your marketing campaigns. These SDKs equip businesses with the needed tools to verify the authenticity of video content in real-time.
-
Real-time verification of video content: Ensure that all video content published or endorsed by your brand undergoes thorough vetting through 3D Liveness Detection. This proactive approach helps maintain brand reputation and authentity by immediately identifying and mitigating any threats posed by deepfake content.
-
Continuous monitoring and improvement: Regularly assess the efficacy of your 3D Liveness Detection implementation, making adjustments as needed to maintain the highest level of accuracy and protection against deepfake videos. Stay updated with the latest advancements in deepfake detection technology to ensure that your prevention efforts remain effective in the face of evolving deepfake capabilities.
Headless Browser Detection and Automation Framework Detection
What is Headless Browser and Automation Framework Detection
Headless browser and automation framework detection are cybersecurity tools used to identify headless browsers, emulators, and automation activities that can be employed in creating and distributing deepfake content. By detecting such activities, digital marketing professionals can prevent distorted market research and the spread of misinformation campaigns that leverage deepfake technology.
How does it work
Headless browser detection involves verifying browser and device characteristics for typical user-agent behavior, essentially distinguishing real users from automated systems. On the other hand, automation framework detection seeks to identify automation signatures in content engagement, revealing patterns that suggest deepfake involvement.
Pros & cons
- Pros:
- Early detection of deepfake-generated content: By spotting the signs of automation and headless browsers, this strategy allows marketers to identify and block deepfake content before it becomes an issue.
- Accurate market research data and campaign insights: Uncovering automation frameworks and headless browsers will lead to a better understanding of user behavior, resulting in more reliable data for marketing initiatives.
- Cons:
- Complex implementation and maintenance: Implementing headless browser and automation framework detection can be challenging, as it requires specialized knowledge, and continuous adjustments to stay ahead of evolving adversarial techniques.
- Possibility of false positives: Any detection strategy relies on accurately identifying suspicious activities. However, due to the complexity and diversity of user behavior, as well as the continuous development of deepfake technology, false positives can occur, leading to legitimate users getting flagged and potentially blocked.
Tactical implementation
To reap the benefits of headless browser and automation framework detection, marketing professionals should consider utilizing libraries like Puppeteer-extra, which provides functionality for detecting headless Chrome browsers. Additionally, they should make use of honeypots, bot traps, and other cybersecurity mechanisms embedded in online content to deter or catch automated systems.
By combining headless browser detection with automation framework identification, digital marketing professionals can better spot the presence of deepfake content on their platforms and act swiftly to limit its potentially damaging effects on their campaigns or brand reputation. However, given the complexity and evolving nature of deepfake technology, they must also remain vigilant and adaptive to stay ahead of this cyberthreat in the long term.
Strategy 5: Behavior Similarity Search
What is Behavior Similarity Search
Behavior Similarity Search is a method of identifying patterns and anomalies in user behavior using AI and behavioral analysis. This technique is particularly useful in the detection and prevention of deepfake-based phishing campaigns within the advertising and marketing industry.
How does it work
Behavior Similarity Search works by profiling user behaviors and establishing baseline patterns for genuine user interactions in various online platforms. By closely monitoring these patterns, the system can then detect deviations and anomalies that suggest the involvement of deepfake-generated content or interactions, such as artificially created testimonials, reviews, or endorsements.
Pros & cons
-
Pros:
- Efficient exposure of deepfake-created testimonials: This method can quickly and effectively identify instances where deepfake technology has been used to create user reviews or endorsements, ensuring that marketing efforts are not compromised by fraudulent content.
- Identifies targeted phishing campaigns: By detecting anomalies in user behavior, Behavior Similarity Search helps pinpoint instances where deepfake-based phishing campaigns are being used to manipulate marketing campaigns or deceive users.
-
Cons:
- High reliance on behavioral data quality: The effectiveness of this method largely depends on the quality of the behavioral data collected. Insufficient or low-quality data may lead to inaccurate detection of deepfake-generated content.
- Computational complexity: Implementing Behavior Similarity Search may require significant computing resources and expertise, which can be challenging for smaller organizations or those with limited technical capabilities.
Tactical implementation
To implement Behavior Similarity Search in your advertising and marketing campaigns, you can follow these steps:
-
Integrate machine learning platforms like TensorFlow or Scikit-learn into your analysis workflow. These platforms will help you build and train AI models that can efficiently analyze user behavior patterns and identify anomalies.
-
Collect extensive behavioral data on user interactions with your advertising and marketing content. This includes information on user click patterns, time spent on the website, the frequency of engagement with different content types, and any other relevant data that can help establish baseline patterns of genuine user behavior.
-
Analyze the collected data using the AI models to detect patterns and anomalies in the user interactions.
-
Regularly monitor and update the AI models to ensure they remain effective at detecting deepfake-generated content. As deepfake technology evolves, it’s crucial to keep your detection methods up-to-date to maintain their accuracy.
-
When an anomaly is detected, you may consider conducting further investigation or adopting additional deepfake detection methods to confirm the presence of deepfake-generated content.
By integrating Behavior Similarity Search into your digital marketing strategies, you can effectively protect your campaigns from the negative impact of deepfake technology, ensuring that your marketing efforts remain genuine and produce successful results.
Final Thoughts and Next Steps
In summary, deepfakes pose a significant threat to the advertising and marketing sectors, with possible consequences ranging from reputational damage to the effectiveness of campaigns. By understanding and employing the top 5 technical tactics - device and browser fingerprinting, facial biometrics, 3D liveness detection, headless browser detection and automation framework detection, and behavior similarity search - digital marketing professionals, advertisers, media practitioners, and AI enthusiasts can mitigate the risks associated with deepfake fraud.
Key takeaways include:
- The need for a proactive approach to deepfake risk management
- Utilizing a combination of technical tactics to comprehensively address various aspects of deepfake fraud
- Constantly updating risk mitigation strategies as deepfake technology evolves
The next steps for marketers and advertisers should be to:
- Assess their existing cybersecurity measures and identify gaps that may be exploited through deepfake fraud
- Review the tactics mentioned above, evaluate which methods are feasible and most suitable for their organization, and integrate these solutions into their marketing platforms
- Regularly monitor for advancements in deepfake technology and adapt their risk management strategies accordingly
By being well-equipped to handle deepfake fraud and staying vigilant on emerging threats in the digital landscape, marketing and advertising professionals can successfully protect their brand reputation, maintain the integrity of their campaigns, and make informed decisions about the type of content they use and endorse.