Safeguard Your Entertainment Platform with Anomaly ML Fraud Detection
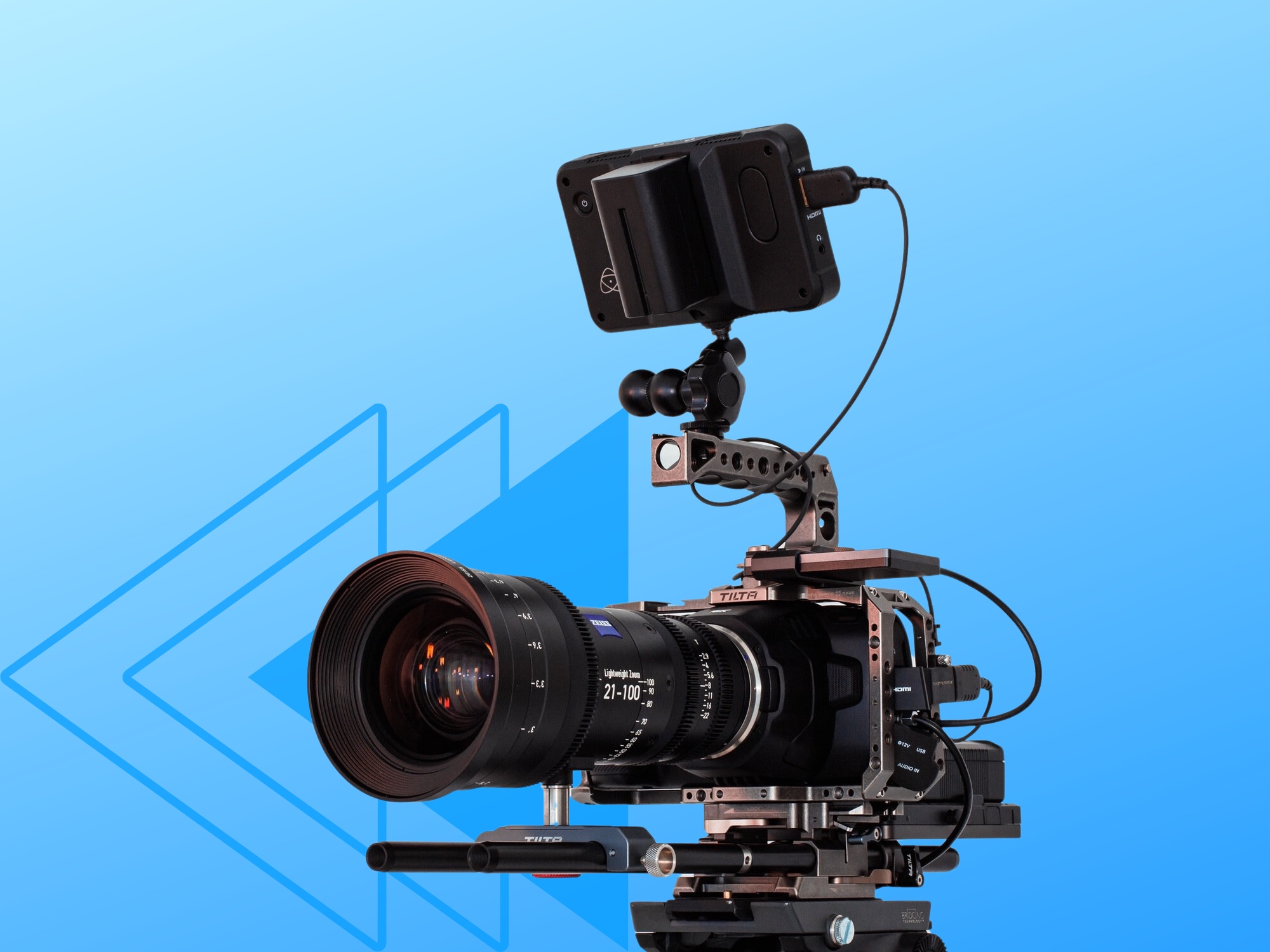
The entertainment and publishing sectors continuously evolve in a digital world, and alongside their innovation, the specter of cybercrime looms. Fraudulent activities, notably fake user registrations, content piracy, and account takeover attempts, threaten to destabilize the pivotal balance between user experience and platform security. The antidote to this malaise comes in the form of Anomaly Machine Learning (ML) - a sophisticated weapon in the cyber arsenal against such nefarious activities.
Anomaly ML stands tall in the modern cybersecurity efforts, deriving its prowess from the ability to learn and predict data patterns. It then flags activities that deviate from the norm, which could potentially be fraudulent. This capability is crucial as the entertainment and publishing industries now demand more robust fraud detection systems that not only react to threats but anticipate and negate them preemptively.
This introduction of Anomaly ML aligns with the goals and challenges of a diverse audience within the entertainment and publishing sectors - from CTOs to product managers, and growth strategists to data scientists. Each seeks solutions that blend seamlessly into their operational fabric, enhancing security measures without trading off the user satisfaction or impeding the organic growth of their platforms. As we delve further, we'll explore how Anomaly ML reconciles the intricate dance between user protection and experience, and why it is increasingly becoming the go-to strategy for fraud prevention within these dynamic industries.
Understanding Anomaly ML in Fraud Detection
Anomaly ML: A Primer
Machine Learning (ML) is a powerful tool in detecting unusual patterns and anomalies that could indicate fraudulent activity. At its core, ML leverages algorithms that enable systems to learn from data, identify patterns, and make decisions with minimal human intervention. Within the realm of fraud detection, anomaly detection capabilities of ML play a pivotal role.
In fraud detection, ML can be implemented through various approaches:
-
Supervised Learning involves training a model on a labeled dataset where the outcomes are known. This method is proficient in recognizing known types of fraudulent transactions.
-
Unsupervised Learning operates on unlabeled data, identifying anomalies based on deviations from what it considers "normal" behavior. This is crucial for detecting new or emerging types of fraud.
-
Semi-supervised Learning combines aspects of both supervised and unsupervised learning, typically using a small set of labeled data alongside a larger set of unlabeled data. It's effective in environments where obtaining a completely labeled dataset is impractical.
With these methodologies, Anomaly ML adapts to the intricate patterns of user behavior in entertainment and publishing platforms, distinguishing between legitimate interactions and potentially fraudulent ones.
Anomaly ML at Work
The implementation of Anomaly ML in fraud detection is a systematic process that involves several critical steps from data acquisition to actionable insights:
-
Data Collection: Accumulating vast amounts of user interaction data, including login frequency, transaction history, and content consumption patterns.
-
Data Processing: Cleaning and preparing the data to ensure that it is suitable for model training. This step might involve handling missing values, removing duplicates, and feature engineering.
-
Model Training: Using the chosen ML framework to train models on historical data, the models learn to identify what normal behavior looks like.
-
Model Deployment: Integrating the trained model into the live environment, where it begins analyzing real-time data.
-
Real-time Analysis: Continuously monitoring user actions on the platform, with the ML model analyzing each event for potential anomalies.
-
Alert Generation: If an anomaly is detected, the model triggers an alert. These alerts can be configured to be as detailed as necessary, providing insights into the nature of the anomaly and suggestions for response.
Through this process, Anomaly ML systems provide entertainment and publishing platforms with the capability to monitor transactions and interactions at a scale and speed that manual monitoring could never match. By analyzing every action in real-time, these systems can detect and flag anomalous behavior that could indicate fraudulent activity, such as abnormal login patterns, suspicious payment transactions, or atypical content access that might suggest content piracy.
The Front Line of Cybersecurity for Publishers & Entertainers
Proactive Defense in Digital Space
For publishers and entertainers, cybersecurity is an ever-present battleground, and Anomaly ML stands as a critical defense mechanism in this digital theater of operations. Machine learning algorithms, with their capacity to identify aberrant patterns and behaviors, offer a proactive approach to thwarting fraudulent activity before it can cause significant damage. Real-time anomaly detection is a game-changer, giving security teams the edge to respond to threats instantly.
Take the example of fake user registration, which not only skews analytics but can also be a gateway for further malicious activities. Anomaly ML can detect unusual sign-up rates or aberrant data entry that deviates from established patterns. Similarly, account takeover attempts – which often follow specific behavioral patterns – can be detected early, preventing unauthorized access and preserving user trust. Cases of early detection are numerous and illustrate the versatility of Anomaly ML in safeguarding business assets and maintaining uninterrupted service to legitimate users.
Battle Against Content Piracy
In the entertainment world, content is king, but it is perennially threatened by piracy. Anomaly ML steps in as a formidable ally, protecting the lifeblood of the industry. By analyzing content access patterns, download behaviors, and distribution channels, Anomaly ML systems can identify potential piracy in progress.
Once Anomaly ML recognizes suspicious behavior—such as a sudden spike in content downloads from an atypical geography or multiple access requests from varied locations—it triggers alerts. This allows the business to take immediate action, such as blocking the user or restricting access. Additionally, pattern recognition capabilities are essential for distinguishing between common sharing behaviors and malicious piracy efforts, thus ensuring that intellectual property rights are preserved without inconveniencing legitimate users. This is particularly relevant as the line between consumers and distributors continue to blur with the advent of social media and user-generated content platforms.
By adopting Anomaly ML for cybersecurity, entertainment and publishing platforms are not only combating fraud but also protecting their revenue streams and the very essence of their creative output. Protecting intellectual property through vigilant, automated systems makes machine learning an indispensable tool in the battle against piracy.
In summary, Anomaly ML provides an advanced, pre-emptive shield against the multifaceted threats faced by publishers and entertainment companies. Its sophisticated pattern recognition empowers these industries to maintain the integrity of their digital spaces and defend against the economic and reputational repercussions of fraudulent activities.
Get started with Verisoul for free
Bridging Growth and Security through Anomaly ML
Cultivating User Trust with Invisible Guardians
In the entertainment and publishing industries, the importance of cultivating user trust cannot be overstated. With fraudsters continually devising new strategies to exploit vulnerabilities, Anomaly ML has emerged as the invisible guardian that operates silently behind the scenes. This advanced technology ensures that security measures do not obstruct the user experience; instead, they bolster the relationship between users and platforms by offering a seamless and secure online environment.
Anomaly ML is engineered to be unobtrusive, integrating with user interactions without introducing friction or delays that can lead to dissatisfaction or abandonment of the platform. By doing so, it allows users to engage with content, make transactions, and explore new services with confidence, knowing that their personal information is protected from fraudulent activity. This reassurance contributes to higher user retention rates, as trust is a key factor that influences user loyalty.
For entertainment platforms and publishers, utilizing Anomaly ML translates into robust security that users can trust, even if they are not aware of the intricate processes working to safeguard their activities. This implicit guarantee of security is critical for platforms that handle large volumes of user data and financial transactions, as it leads to a reputation of reliability that is invaluable for long-term growth.
Scaling Safely
The dynamic nature of the entertainment and publishing sectors demands solutions that can scale with the growth of the platform. Anomaly ML addresses this need by providing scalable fraud detection that adapts to increasing volumes of user activity and data without compromising performance. The integration of Anomaly ML into existing infrastructure is a key concern that product managers and developers face, seeking to avoid any disruption to services or user experience.
Scalability is intrinsic to Anomaly ML algorithms, which are designed to handle large datasets and swiftly adjust to patterns in user behavior as a platform expands. The adoption of Anomaly ML does not require an overhaul of current systems; rather, it integrates with existing protocols to enhance their capabilities. This seamless integration ensures that security measures grow in tandem with the platform, maintaining a consistent level of defense against fraud without impeding functionality or speed.
By enabling safe scaling, Anomaly ML provides a double-edged advantage: it underpins ongoing security efforts, and it equips platforms to manage the influx of new users and the complexities that come with larger datasets. For growth managers and marketers, the result is a clear path to expanding their user base while maintaining a secure environment—an essential balance in the competitive landscape of digital entertainment and publishing.
With Anomaly ML, technical decision-makers can rest assured that their security posture is fortified, and their platform is equipped to handle growth without exposing the business or its users to increased fraud risks. Integration concerns are addressed by the technology's compatibility with existing infrastructure, ensuring that scaling up is a safe and smooth process.
Limitations and Overcoming Hurdles
The Challenge of False Positives/Negatives
In the realm of Anomaly ML fraud detection, false positives and negatives pose significant challenges. When an anomaly detection system incorrectly flags legitimate activity as fraudulent, it results in a false positive. Conversely, a false negative occurs when actual fraudulent activity goes undetected. Both can undermine the efficiency of a cybersecurity strategy.
- Data Quality: The success of Anomaly ML is heavily dependent on the quality of the data fed into the system. Inaccurate or incomplete data sets can train models to make unreliable predictions.
- Continuous Refinement: It is crucial to continually tweak and refine detection algorithms to minimize errors. Regular audits of false positives can help in adjusting the sensitivity of the system.
- User Feedback Loop: Implementing a system where user feedback is taken into account can help improve the accuracy of fraud detection over time.
- Cross-Verification Methods: Layering multiple verification methods can help balance out the inaccuracies of any single mode of detection.
By embracing a comprehensive approach to data management and analysis, companies can significantly reduce the incidences of false alerts, thus preserving the user experience and focusing security efforts where they are most needed.
Navigating the Evolving Fraud Landscape
With fraud tactics becoming more complex and sophisticated, Anomaly ML models need to be dynamic enough to adapt to new patterns of fraudulent behavior. Here's how businesses can maintain sharp defenses:
- Ongoing Training: Machine learning models are not a one-and-done solution. They require ongoing training with new and relevant data to stay effective. Regular updates to the model with novel fraud instances are crucial.
- Human Oversight: The subtleties of human behavior are difficult to fully capture with algorithms. Hence, skilled fraud analysts should oversee the ML outputs to provide context that the model may miss.
- Cross-functional Teams: Creating specialized teams combining AI experts with fraud analysts can foster an environment where machine efficiency and human intuition work in concert.
- Threat Intelligence Sharing: Engaging in industry-wide collaborations to share insights on newly emerging fraud tactics can help in training Anomaly ML models to recognize and flag these patterns.
By ensuring machines and humans work together and by investing in the ongoing development of ML models, the entertainment industry can continue to stay ahead in the battle against cybercrime and ensure a secure experience for all users.
Final Thoughts and Next Steps
As we navigate the complex landscape of the entertainment and publishing industries, the battle against fraudulent activity remains a dynamic and persistent challenge. Anomaly Machine Learning (Anomaly ML) stands out as a technological beacon, offering a sophisticated line of defense to protect digital assets, personal data, and overall brand integrity.
-
Strategic Technology Adoption: It's imperative for industry leaders to view Anomaly ML not just as a tool, but as a strategic asset. Its integration within cybersecurity initiatives should be deliberate, aimed at reinforcing the robustness of fraud detection frameworks.
-
Ongoing Vigilance: Given the erratic nature of cyber threats, maintaining an adaptive stance is crucial. Continuous model training, regular data assessment, and incorporation of new threat intelligence are essential practices that should be institutionalized within operational protocols.
Next Steps for Your Platform
-
Assess Current Fraud Detection Systems: Audit existing security infrastructure to identify potential gaps that Anomaly ML could fill. This will also help to quantify the impact of Anomaly ML integration on overall cybersecurity efficacy.
-
Pilot Anomaly ML Initiatives: Engage in pilot programs that will allow for real-world testing of Anomaly ML systems. Use this opportunity to gauge scalability, user experience impact, and the accuracy of threat detection.
-
Educate and Train Your Team: Invest in the ongoing education of technical teams and stakeholder groups to ensure a comprehensive understanding of Anomaly ML. Knowledge dissemination is key, from the intricacies of model creation to the nuances of threat response protocols.
-
Monitor Regulatory Developments: Stay abreast of global privacy and cybersecurity regulations to ensure compliance and to anticipate how evolving laws may shape the deployment of Anomaly ML technologies.
Looking to the Future
The evolution and future outlook of Anomaly ML in cybersecurity are predicated on enhanced computational models, richer datasets, and broader inter-industry collaboration. The sophistication of fraudulent schemes will inevitably progress, but so too will the capacity of Anomaly ML to detect and neutralize threats before they escalate into security incidents. As we press forward, engaging with Anomaly ML is less of an option and more of a requisite for preserving the security and continued prosperity of entertainment and publishing platforms.