SaaS CTOs Leverage Anomaly ML for Enhanced Fraud Prevention
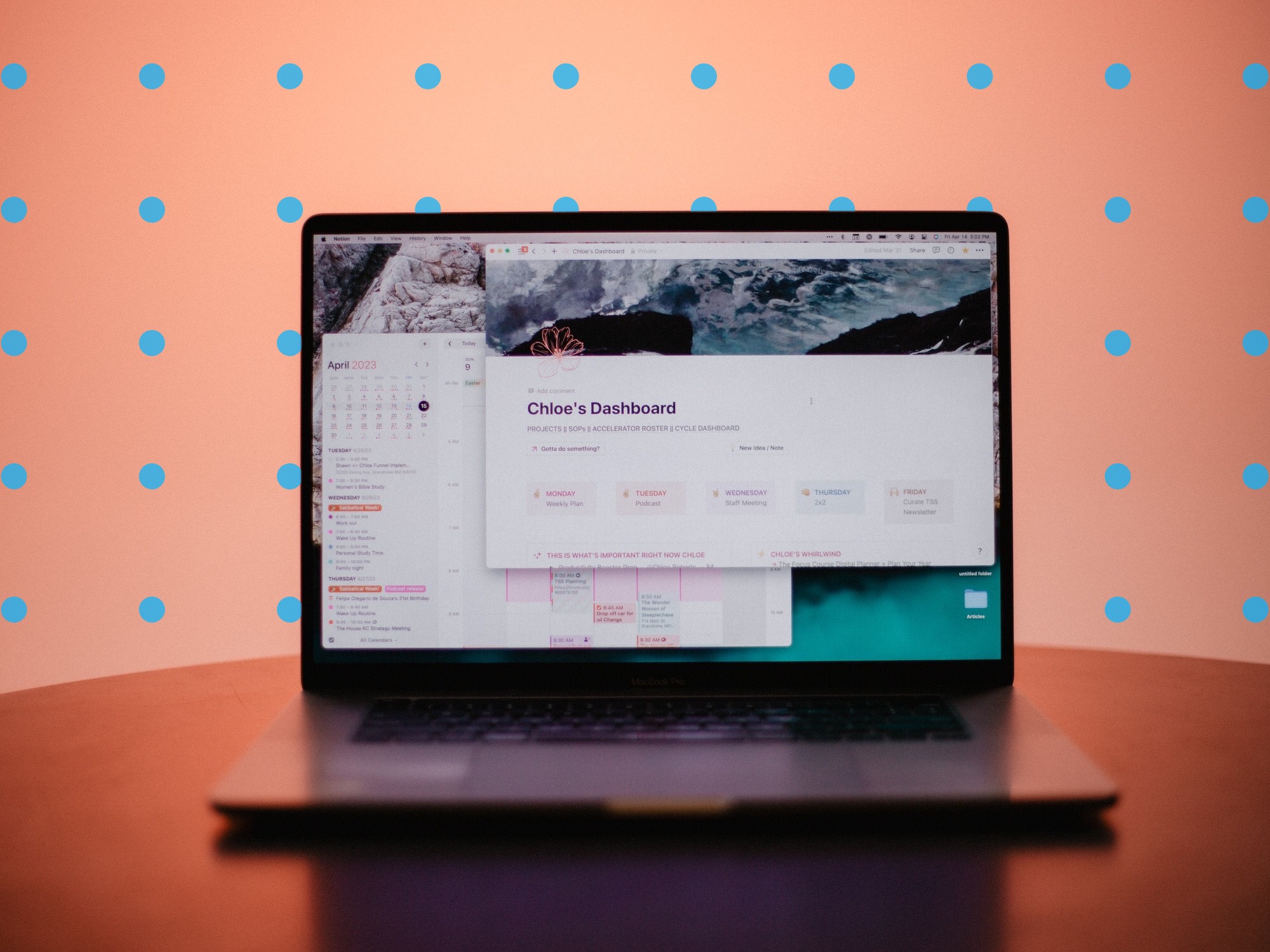
Fraud prevention has emerged as an indispensable pillar for SaaS companies to protect their ecosystems and maintain a loyal user base. Chief Technical Officers and data-driven teams face the unending challenge of staying ahead of sophisticated fraudsters. Here, anomaly detection machine learning (ML) offers a compelling solution by flagging irregular patterns that deviate from expected user behavior, signaling potential fraudulent activities.
Understanding and responding to these threats is not optional; it's a critical component of a company's survival and success. A security breach can erode user trust—a commodity more valuable than ever in the digital age—damaging a company's brand and bottom line. As CTOs, product managers, and cybersecurity experts scrutinize their security infrastructure for gaps, incorporating robust, scalable ML solutions for fraud detection is top of mind. By leveraging the power of anomaly detection ML, SaaS organizations ensure their platforms' integrity and fortify their reputations.
This blend of technology and necessity breeds an innovative environment where user trust and the smooth functioning of platforms are seen as two sides of the same coin. Anomaly detection ML is pivotal in forging secure SaaS applications that users can rely on, fostering a safe space for digital interactions and transactions to flourish. Implementing scalable security measures not only protects but also serves as a growth engine, underpinning the user confidence that fuels SaaS expansion.
Understanding Anomaly Detection ML in SaaS
Defining Anomaly Detection and its role in Fraud Prevention
Anomaly Detection is a critical component of cybersecurity, pivotal for SaaS platforms that handle sensitive user data and transactions. Essentially, Anomaly Detection Machine Learning (ML) algorithms are designed to identify patterns that do not conform to expected behavior—flagging them as potential fraud. This technology is pertinent to SaaS CTOs who seek a proactive approach to preserving their platforms' integrity.
When it comes to differentiating between ML models, there are three main types employed in Anomaly Detection: Supervised, Semi-supervised, and Unsupervised. Supervised ML models are trained on historical data labeled as normal or anomalous. In contrast, Semi-supervised ML models are trained on a data set where only the normal cases have been labeled. Lastly, Unsupervised ML models identify anomalies without any labeled training data, instead relying on the assumption that the majority of the instances in the dataset are normal. Each type has different applications and efficacy in the context of fraud detection in SaaS environments.
How Anomaly Detection ML unpacks user behavior to identify fraud
At the core of Anomaly Detection is behavior analytics—ML algorithms analyze user behavior and identify activity that strays from the norm. Large-scale SaaS platforms benefit immensely from this technology due to the high volume of user interactions; manual monitoring at this scale is practically infeasible.
By utilizing statistical models, ML can assess user actions within the context of historical data. More sophisticated neural networks and deep learning architectures offer even greater depth in analyzing intricate patterns and sequences in behavior that humans might miss. These technologies—particularly Recurrent Neural Networks (RNN) and Autoencoders—excel at picking up on subtle anomalies that could indicate sophisticated fraud schemes, such as identity theft or financial fraud.
For SaaS CTOs and cybersecurity experts, equipping their security frameworks with Anomaly Detection ML means having an automated, vigilant sentinel. This sentinel can process vast amounts of data to discern legitimate user actions from potentially fraudulent ones. Moreover, their capabilities adapt over time with each new data input, refining their accuracy in spotting fraudulent incidents.
Bullet-list highlights of this section:
- Anomaly Detection ML is critical for identifying fraudulent activity in SaaS platforms.
- ML models can be Supervised, Semi-supervised, or Unsupervised, each with unique applications in fraud detection.
- Behavior analytics powered by ML examines user behavior, leveraging statistical models, neural networks, or deep learning to identify anomalies.
- Technologies like RNNs and Autoencoders detect subtle patterns indicating fraud, beyond the reach of manual monitoring.
- Anomaly Detection ML acts as an automated system that continuously enhances its fraud detection capabilities over time.
Advantages of Implementing Anomaly Detection ML
Scalability: Tailoring Fraud Detection to SaaS Dynamics
One of the principal benefits of anomaly detection machine learning (ML) in Software as a Service (SaaS) is its scalability. AI-driven systems are incredibly adept at analyzing large datasets that SaaS companies accumulate as their user base grows. These systems are particularly powerful in real-time processing, crucial for dynamic SaaS operations where user interactions are constant and immediate response is essential.
- ML models can scale with the user base, adapting to increased loads without loss of performance.
- Real-time processing allows for the identification of anomalies as they occur, providing opportunities for instant mitigation of potential fraud.
- Scalability ensures that as a SaaS platform expands, its capacity to detect and prevent fraud remains uncompromised and efficient.
Enhanced Accuracy: Tuning ML for Precise Fraud Identification
The precision of anomaly detection ML is paramount to minimizing false positives and false negatives—where legitimate users are flagged, or fraudulent activities are missed. Enhanced accuracy in fraud detection reinforces trust in the user experience; genuine users are less likely to encounter friction, while fraudsters find it harder to bypass security measures.
- Techniques such as feature engineering, model training, and cross-validation help fine-tune ML models to the unique fraud patterns of each SaaS platform.
- Implementing a combination of supervised and unsupervised learning can improve detection accuracy by leveraging both labeled data and identifying new, unforeseen fraud tactics.
Adaptive Learning: Keeping Up with Evolving Fraud Tactics
Fraudsters constantly evolve their tactics, which means static security systems quickly become outdated. Anomaly detection ML is inherently adaptive; it learns from new data and adjusts to new patterns of fraud.
- Continuous learning allows ML models to stay ahead of fraudsters by evolving with the changing tactics.
- ML can identify complex fraud schemes that traditional rule-based systems may overlook.
Automation: Streamlining Fraud Detection for Operational Efficiency
Automation stands out as one of the most clear-cut advantages of implementing ML in fraud detection. It reduces the need for manual review and enables SaaS companies to reallocate human resources to tasks that require human judgment and creativity.
- Automated alerts and actions can be configured to respond to identified threats, reducing the response time and the potential damage of fraudulent activities.
- The overall efficiency of the operational process is improved, as automated systems handle the labor-intensive tasks of data analysis and fraud detection, freeing teams to focus on more strategic initiatives.
- By automating repetitive tasks, anomaly detection ML not only enhances fraud prevention measures but also contributes to the overall satisfaction and retention of security teams, who can engage in more meaningful work.
In integrating anomaly detection ML into their fraud prevention strategies, SaaS CTOs ensure their systems remain robust against the constantly shifting landscape of cybersecurity threats. This secures their operations, engenders customer loyalty, and ultimately supports sustainable growth and success in the competitive SaaS market.
Get started with Verisoul for free
Challenges and Considerations in Anomaly Detection ML Deployment
The Data Quality Imperative
For SaaS CTOs, the foundation of effective anomaly detection ML is data quality. High-quality, labeled datasets are not just a requirement but an absolute necessity for training sophisticated machine learning models. In the cybersecurity realm, the precision of fraud detection directly correlates to the integrity and granularity of the data at hand. A robust dataset ensures that the ML models can learn from accurate examples of both legitimate behavior and fraudulent patterns, minimizing the instances of false positives and false negatives.
However, data collection is often rife with challenges, including inconsistency, incompleteness, or noise within the data. CTOs must thus prioritize data cleaning and pre-processing to develop a dataset that can power an effective ML-driven fraud prevention system.
The Complexity and 'Black Box' Dilemma
Anomaly detection models, particularly those built on complex algorithms like neural networks, can suffer from the 'black box' dilemma. Despite their capability to identify nuanced patterns of fraud, explaining the decision-making process of these models can be difficult. Transparency in ML is critical for trust within an organization, as well as for regulatory compliance.
CTOs and security teams should consider ML interpretability tools and frameworks that help decipher model predictions. This transparency not only builds confidence in ML outputs but also assists in fine-tuning the models for better accuracy.
Balancing Cost and Performance
Investing in anomaly detection ML involves consideration of both the immediate costs and the potential return on investment (ROI). For SaaS businesses, the upfront expense includes not only the development or purchase of machine learning solutions but also the computation power to train and run these models.
CTOs must balance the cost with performance benefits, such as reducing the need for large fraud analysis teams and decreasing the financial losses due to fraud. When managed effectively, the savings and added value brought by ML can far exceed the initial investment.
Continuous Evolution: The Need for Ongoing Model Maintenance
Deploying an ML model for anomaly detection is not a set-and-forget solution. Cybercriminals are continually developing new tactics, and the ML models must evolve to keep pace. Ongoing maintenance and regular updates to the ML model are critical to ensure long-term effectiveness.
This means establishing processes for continuous learning, wherein the system is regularly fed new data to refine its detection capabilities. SaaS companies must also maintain a dedicated team or service to monitor the health and performance of ML models, ensuring that they remain up-to-date with the latest fraud trends and techniques.
Enhancing User Trust with Secure Environments
Building a secure environment is imperative for SaaS companies not only to protect their assets but also to solidify user trust. Cybersecurity threats, if not mitigated, can lead to breaches that might compromise user data and erode the credibility of a service provider. By employing Anomaly Detection ML, SaaS CTOs can proactively address potential threats, thereby enhancing the overall trust that users have in the platform. The benefits are manifold:
- Immediate Detection and Response: Anomaly ML quickly identifies abnormal patterns, enabling swift countermeasures against potential fraud.
- User Confidence: Customers feel safer knowing that their data is protected by advanced, adaptive security measures.
- Compliance Assurance: Staying ahead of compliance requirements by proactively countering fraud helps reinforce regulatory trust.
A user-centric growth model leverages this trust, leading to increased user retention and referrals, both of which are crucial for SaaS platforms.
The Interplay Between Anomaly Detection and User Experience
The integration of Anomaly Detection ML should not compromise user experience (UX). A balance must be struck; powerful fraud prevention algorithms run in the background, while front-end UX remains user-friendly and inviting. SaaS companies should strive to:
- Minimize Intrusion: Use Machine Learning to conduct security checks without disrupting user interaction.
- Smooth Onboarding: Ensure security processes during account creation are streamlined and non-invasive.
- Transparent Security: Educate users on the benefits of security measures to gain their support and cooperation.
These strategies underscore that strong security and enjoyable user experiences are not mutually exclusive; rather, they complement each other in creating robust SaaS platforms.
Growth Metrics Integrity: Cutting Through the Noise of Fraudulent Data
The integrity of growth metrics is crucial for assessing a SaaS company’s health and directing its strategies. Anomaly ML serves as a gatekeeper, distinguishing between genuine user interaction and fraudulent activity. This accuracy in metrics allows companies to:
- Assess True Growth: With clean data, SaaS entities can accurately measure user acquisition and retention.
- Tailor Marketing Efforts: Marketing teams depend on precise data to optimize campaigns and user outreach.
- Invest Strategically: Companies can allocate resources confidently when they are assured of the legitimacy of their user base.
By ensuring the authenticity of growth metrics, Anomaly Detection ML allows SaaS businesses to chart a course for sustainable expansion, free from the distortion of fraudulent interferences.
Final Thoughts and Next Steps
As we close the dialogue on Anomaly Detection ML, it's apparent that its integration is a critical component for the SaaS industry’s battle against fraud. The cognitive leap from legacy systems to intelligent, adaptive ML models represents more than an upgrade—it’s a transformation in how we understand and respond to threats.
- Importance of Anomaly Detection ML: Staying at the forefront of fraud prevention is a must for protecting a company's bottom line and user trust. It’s not just about detecting anomalies; it’s about fostering an environment where security and user experience go hand in hand.
- Addressing Challenges: Committing to overcome obstacles like data quality and model complexity will unlock further potential for Anomaly Detection ML. This includes a transparent approach to the 'Black Box' of ML, ensuring interpretability and trust in the systems we deploy.
- Taking Action: For actionable next steps, SaaS CTOs and security teams should:
- Conduct a thorough assessment of current fraud prevention measures.
- Explore case studies and benchmarks in Anomaly Detection ML.
- Engage with ML experts or consider building a specialized in-house team.
- Prioritize the integration of ML systems that can scale with your growing data streams and user base.
Remember, the road to enhanced fraud prevention is iterative and requires ongoing attention. An anomaly detection system that's exemplary today might need fine-tuning tomorrow as threats evolve. However, through a strategic blend of expert knowledge and continuous innovation, SaaS organizations can not only protect themselves more effectively but also establish a competitive edge in an ever-changing digital landscape.
- Embracing Innovation: In a digital epoch characterized by rapid change, your commitment to innovation defines success. Integrating Anomaly Detection ML is not merely an option but a necessity for SaaS companies seeking robust growth and exemplary user trust.
Looking ahead, the intersection of anomaly detection and other emerging technologies like blockchain and quantum computing can only expand the horizon for cybersecurity in SaaS. The future belongs to those who are proactive, resilient, and ever-vigilant in the face of new types of cyber threats.