Preventing Deepfake Disruption in Sharing Economy Platforms
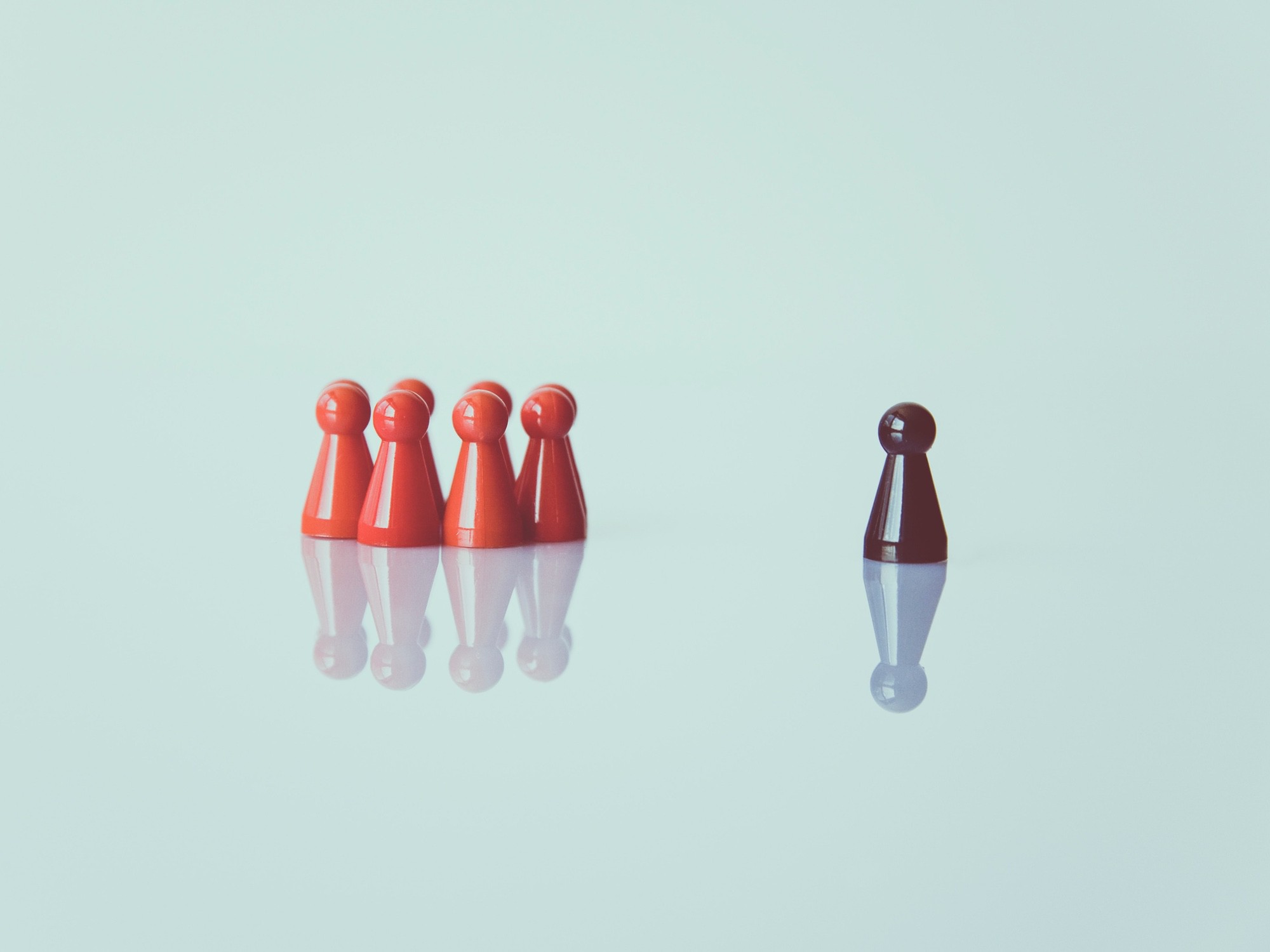
Online marketplaces and sharing economy platforms depend on the authenticity of user profiles and content to establish trust and maintain security. The rise of deepfakes, a sophisticated form of artificial media created using advanced machine learning algorithms, poses significant risks to the integrity of these platforms. For decision-makers such as CTOs, CISOs, Product Managers, and Developers, understanding the implications of deepfake technologies and implementing strategies to detect and combat related fraud is essential for sustaining platform growth and user confidence.
As deepfakes become increasingly difficult to identify, they pose an array of unique challenges for our audience. These decision-makers need to anticipate potential threats and develop multi-faceted approaches to ensure that user experiences remain authentic and secure. This may include investing in advanced technologies, refining detection methods, and partnering with industry experts to stay informed on emerging trends and best practices against deepfake-related fraud.
By prioritizing efforts to detect and prevent deepfake disruption, these professionals can effectively protect their digital ecosystems from a growing threat landscape and ensure user trust in their platforms. In order to achieve this, a cohesive understanding of deepfake technologies, their potential impact, and practical fraud detection and prevention methodologies is crucial. This article aims to provide our audience with valuable insights, strategies, and recommendations to address this challenge head-on and secure their platforms against deepfake-driven deception.
Understanding Deepfakes and Fraud Techniques
Defining Deepfakes and their Underlying Technologies
Deepfakes refer to manipulated images, videos, or audio recordings that leverage artificial intelligence (AI) technologies, such as Generative Adversarial Networks (GANs), to create realistic but fake content. By training GANs on vast datasets of images, videos, or audio recordings, these neural networks can convincingly generate synthetic media that resembles real content. As the technology becomes more advanced, the quality of deepfakes improves, making it harder for humans and software to distinguish them from genuine content.
Fraud Techniques Used by Bad Actors
Deepfake technology can serve as a powerful tool for bad actors seeking to perpetrate various types of fraud on sharing economy platforms. Some of the prevalent fraud techniques involving deepfakes include:
-
Video manipulation: Fraudsters can use deepfakes to create realistic videos of people saying or doing things they never actually did. These manipulated videos can be used to deceive users, damage reputations, or manipulate public opinion.
-
Voice synthesis: By leveraging deepfake audio technology, bad actors can generate synthetic voice recordings that sound like a real person. These fake voices can be used in scam phone calls or to impersonate individuals in voice-based authentication systems.
-
Social engineering: Deepfakes allow fraudsters to create compelling fake personas that can be used for social engineering attacks. By impersonating executives, employees, or vendors, bad actors can manipulate their targets into taking actions that would benefit the attacker (e.g., transferring funds or disclosing sensitive information).
-
AI-driven automation: With the increasing sophistication of deepfake technology, fraudsters can now employ AI-driven automation to create large-scale attacks on sharing economy platforms. This enables them to generate and distribute deepfakes at an unprecedented scale, making detection even more challenging.
The Increasing Sophistication of Deepfake-Related Frauds
The rapid advancements in deepfake technologies and the democratization of AI tools have led to a significant increase in the sophistication of deepfake-related frauds. These frauds pose a significant risk to sharing economy platforms and their users, as they can lead to financial losses, reputational damage, and a loss of trust in the platform's ability to ensure user authenticity.
For our target audience, including CTOs, CISOs, Product Managers, and Developers, it is critical to understand these deepfake fraud techniques, stay informed about emerging trends, and adapt their strategies and resources accordingly to protect their platforms and user communities.
Challenges and Goals in Detecting and Preventing Deepfake Fraud
Ensuring User Authenticity
One of the primary goals of our audience is to guarantee user authenticity on their platforms, ensuring that every user is genuine and accurately represented. By protecting user identity and information, sharing economy platforms can build trust with their users while simultaneously discouraging fraudsters from exploiting their platforms using deepfake technologies.
Strengthening Security Measures
Decision-makers must prioritize strengthening security measures on their platforms to mitigate deepfake-related fraud risks. This involves the continuous development and implementation of advanced technologies that can quickly detect and address deepfake attacks. By maintaining robust security systems, companies can prevent unauthorized access to sensitive user data and protect their users from deepfake-driven scams.
Enhancing User Experience
Another goal for our target audience is to provide an exceptional user experience on their platforms. This includes ensuring that all user interactions are authentic while keeping the onboarding and verification processes as frictionless as possible. As deepfakes continue to evolve, it becomes even more critical for sharing economy platforms to balance user experience with stringent security measures to maintain user trust and satisfaction.
Staying Informed about Deepfake Trends and Threats
To effectively combat deepfake-related fraud, stakeholders must stay informed about the latest deepfake trends and threats. This allows them to proactively adapt their platforms to address emerging deepfake challenges and implement necessary countermeasures.
Rapid Advancements in Technology
One of the key challenges faced by industry professionals in detecting and preventing deepfake fraud is the rapid advancement of technology. As deepfake techniques continue to improve, it becomes increasingly difficult for sharing economy platforms to differentiate between real and synthetic media, making it crucial for developers and security experts to stay ahead of the curve.
High-Quality Deepfakes
The proliferation of high-quality deepfakes has made it easier for bad actors to create convincing forgeries that can bypass traditional detection methods. Sharing economy platforms must stay vigilant and invest in advanced deepfake detection tools to protect their users and maintain the integrity of their ecosystems.
Inadequate Training Data
To effectively combat deepfakes, security algorithms require vast amounts of training data. However, acquiring a diverse dataset that represents various situations, demographics, and factors is often challenging. Moreover, as deepfake technology evolves, the need for updated training data becomes increasingly critical.
Resource Allocation and Constraints
Sharing economy platforms often face constraints in resources and budget allocation, making it difficult for them to allocate sufficient resources for research and development in deepfake detection and prevention. These constraints may lead to suboptimal solutions that fail to address the evolving threats and sophisticated techniques used by bad actors.
Get started with Verisoul for free
Best Practices for Detecting and Preventing Deepfake Fraud
In this section, we will provide actionable recommendations for decision-makers and stakeholders within sharing economy platforms and online marketplaces to effectively detect and prevent deepfake fraud. By implementing these strategies, platforms will be better equipped to maintain user trust, mitigate security risks, and foster a secure and authentic digital ecosystem.
Utilizing Advanced AI and Machine Learning Techniques for User Verification
One of the key methods to counter deepfake fraud is by employing AI-based user verification techniques. These systems are designed to verify users’ identity through advanced facial recognition, biometric analysis, and liveness detection—ensuring that real users, not deepfake avatars, are accessing the platform.
Some best practices for AI-driven user verification include:
- Implementing liveness detection solutions that require users to perform specific actions in real-time, like blinking or smiling, to prove their authenticity
- Leveraging biometric data (such as facial landmarks, fingerprints, and other unique traits) for identity verification
- Continuously updating machine learning models with new deepfake samples to train the system in detecting the latest deepfake techniques
Developing and Refining Deepfake Detection Algorithms
Actively working on developing and refining deepfake detection algorithms is crucial to staying one step ahead of bad actors. New research and advancements in deepfake detection should be incorporated into these algorithms regularly, enabling your platform's security infrastructure to effectively identify and block deepfake-related fraud.
Some tips for improving deepfake detection algorithms include:
- Conducting research to identify the telltale signs of deepfake videos, such as inconsistencies in lighting or shadows, and unusual facial movements
- Leveraging AI and machine learning solutions that analyze and identify patterns in audiovisual content to differentiate real users from manipulated content
- Collaborating with academia and cybersecurity experts for the latest advancements in deepfake detection algorithms
Implementing Multi-Layered Security Protocols
Adopting a multi-layered approach to security is essential in combating deepfake fraud. By combining multiple security measures, platforms can make it increasingly challenging for bad actors to breach their defenses.
Consider implementing the following:
- Two-factor authentication (2FA) to add an extra layer of security to user login processes
- Risk-based authentication, which adjusts the level of required authentication based on the user's behavioral patterns and risk profile
- Encrypted data storage and communication systems to protect sensitive user information from unauthorized access
Collaborating with Researchers and Industry Experts on Emerging Trends and Detection Methods
Finally, staying informed about emerging trends and detection methods is vital in the fight against deepfake fraud. Regular collaboration with researchers, cybersecurity firms, and industry experts will provide valuable insights and access to cutting-edge detection solutions.
Keep in mind the following steps to foster a collaborative approach:
- Attend industry conferences, webinars, and workshops to learn about the latest trends and advancements in deepfake detection and prevention
- Engage in knowledge-sharing programs and partnerships with other companies, research institutions, and cybersecurity firms
- Subscribe to relevant publications and newsletters to stay updated on new developments and case studies related to deepfake fraud and detection.
Case Studies of Successful Deepfake Fraud Detection and Prevention
To give more context and practical understanding on how various organizations are implementing successful deepfake fraud detection and prevention measures, let's dive into a couple of real-world case studies. These cases demonstrate key takeaways and lessons learned, which can be applied to our target audience's efforts in ensuring user authenticity and strengthened security measures.
Case Study 1: Siwei Lyu and the Consistent Defect Detection Algorithm
Siwei Lyu, a computer science professor at the University at Albany, along with his team, developed an innovative deepfake detection algorithm that relies on the observation of eye blinking behavior. They discovered that deepfake videos often exhibit a lack of consistent eye blinking. This is due to the fact that many training datasets for GANs used in creating deepfakes contain images of people with their eyes open.
Lyu's team developed an AI algorithm to detect this anomaly in videos by monitoring eye blinking patterns. The technique has shown a high success rate in identifying deepfake videos. This approach offers valuable insights to our target audience on the importance of:
- Focusing on subtle behavior patterns that could be markers for synthetic content
- Implementing AI-driven techniques to detect these markers in user-generated content on sharing economy platforms
Case Study 2: Deeptrace and the Multi-faceted Approach
Deeptrace, an Amsterdam-based cybersecurity company, has developed cutting-edge deepfake detection solutions using a combination of machine learning algorithms, computer vision, and biometric identification techniques. Their software analyzes videos and images for minute inconsistencies that suggest manipulation or generation by GANs.
Notable examples of inconsistencies include unnatural lighting, changes in skin texture, and discrepancies in facial expressions. Deeptrace's sophisticated multi-faceted approach demonstrates the following key takeaways:
- Combining different detection techniques can deliver stronger and more reliable results in identifying deepfakes
- Consistently investing in research and development to stay up-to-date with deepfake technology and its advancements
Case Study 3: Jigstack and the WoSign Verification System
Jigstack, a decentralized autonomous organization (DAO), utilizes a blockchain-based verification system called WoSign for ensuring user authenticity. By using blockchain technology, Jigstack can verify the identity of users on their platform, preventing bad actors from using deepfakes to misrepresent themselves. The solution demonstrates that:
- Exploring non-traditional technologies such as blockchain can offer alternative and reliable methods for user authentication
- Integrating multi-layered security protocols in sharing economy platforms can help minimize the impact of deepfake-related fraud
In conclusion, these real-world case studies showcase the importance of developing and refining deepfake detection algorithms, collaborating with researchers and industry experts, and staying informed about deepfake trends and threats. By taking a proactive approach to addressing deepfake-related fraud, decision-makers within growing, contemporary, and modern companies can ensure robust security measures and enhanced user trust on their platforms.
Final Thoughts and Next Steps
As deepfakes become increasingly sophisticated and widespread, their potential to disrupt online marketplaces and sharing economy platforms can no longer be ignored. Decision-makers and stakeholders involved in platform security and user authenticity must remain vigilant to stay ahead of this evolving threat.
To ensure continued success, it is crucial to:
- Stay informed about the latest advances in deepfake technology and their implications for your platform
- Implement advanced solutions, such as AI and machine learning, to enhance user verification, detection capabilities, and overall platform security
- Adopt multi-layered security protocols that strengthen existing measures while accommodating potential deepfake-related risks
- Collaborate with researchers and industry experts to share knowledge and resources, stay updated on emerging trends, and develop more effective detection methods
Taking these proactive steps will not only mitigate the impact of deepfake-related fraud on your platform but also reinforce user trust and ultimately contribute to your platform's growth.
As the battle against deepfakes continues, it is important to keep evolving and adapting your security strategies. By staying abreast of the latest trends, embracing advanced technological solutions, and fostering a collaborative industry environment, you can effectively fortify your platform against deepfake disruption while concurrently safeguarding user trust and authenticity.