Maximize Ad Spend ROI with Anomaly ML Fraud Detection
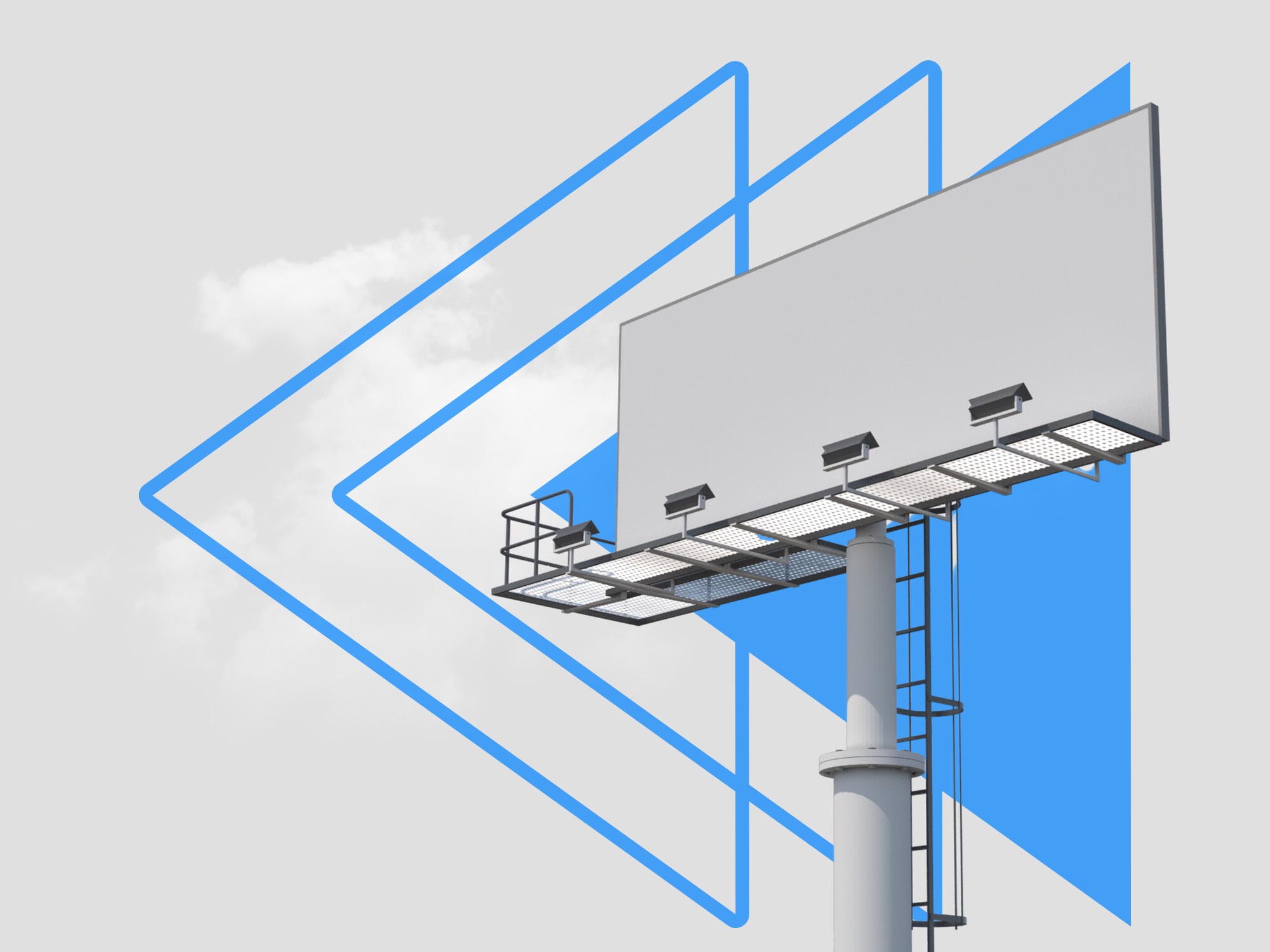
Digital advertising is a burgeoning field, ripe with opportunities but equally vulnerable to fraudulent activities. The dynamic nature of digital platforms means that with every click or conversion, there's a risk of deception that can erode marketing budgets and distort analytics. For marketing professionals, ensuring that each interaction represents a genuine user interest isn't just about authenticity; it's critical for realizing the true return on investment that marketing initiatives promise.
The stakes are high in online marketing; fraudulent clicks, bot traffic, and fake user registrations can inflate metrics and deplete funds, leading to poor decision-making and wasted ad spend. In this intricate digital landscape, where precision is key, Anomaly Machine Learning (Anomaly ML) emerges as a lighthouse for navigating the tricky waters of advertising fraud. This cutting-edge approach to fraud detection can discern irregular patterns amidst a sea of data, helping to shield campaigns from the financial drain and skewed analytics caused by inauthentic activities.
By harnessing the power of Anomaly ML, those in charge of steering digital campaigns—be it CMOs or product managers—can take a proactive stance against fraud. This technology not only enhances the security of marketing initiatives but also refines the accuracy of campaign insights, crucial for the AdTech and MarTech arenas. Data scientists and growth hackers alike will appreciate the robust, data-driven means to a secure and optimized online marketing strategy that Anomaly ML provides.
Understanding Anomaly ML in Ad Fraud Prevention
Fundamentals of Anomaly ML for Marketers and Technologists
Machine Learning (ML) in advertising, particularly Anomaly ML, has become an invaluable tool for marketers looking to combat fraud and safeguard their digital campaigns. At its core, Anomaly ML applies advanced algorithms to detect irregular patterns that deviate from normal behavior within large sets of user data. By continuously learning from new data, these models become adept at identifying fraudulent activities, such as fake clicks or artificial traffic generated by bots.
When integrating Anomaly ML into a marketing strategy, it's important to understand that it works by establishing baseline patterns of user engagement, including click-through rates, conversion rates, and browsing behavior. Then, it uses unsupervised learning algorithms to monitor live data for deviations from these patterns. This kind of detection is critical, as it alerts digital marketers to suspicious activities that could indicate ad fraud. Once flagged, marketers can further investigate or automatically block these anomalies, thereby ensuring only genuine interactions are counted towards their advertising spend.
Navigating Through Big Data with Machine Learning
Dealing with the vast amount of data generated by digital advertising campaigns can be overwhelming. The journey from data collection to real-time anomaly detection begins with the aggregation of user interactions across various platforms. Structured data is fed into Anomaly ML models, which then analyze this information in real time to identify potential fraud.
Several algorithms play pivotal roles in anomaly detection, each offering unique advantages depending on the nature of the data and the specific type of fraud being addressed. For example:
- Cluster analysis algorithms identify groups of similar data points and can flag anomalies that do not fit into any cluster.
- Neural networks, inspired by human brain function, are particularly effective in learning complex, nonlinear relationships in data and identifying nuanced patterns of fraud.
- Support vector machines create a boundary between normal behavior and anomalies, working well in scenarios with clear margins of separation.
Moreover, decision tree models can break down the data into simpler decision nodes, making them a strong choice for interpretability, which is often as crucial as detection. Similarly, random forest models aggregate the results of multiple decision trees to improve detection accuracy, reducing the risk of overfitting which is common in high-dimensional data.
Each of these algorithms contributes to a robust Anomaly ML framework capable of sifting through the noise to detect fraudulent activities that could otherwise drain ad budgets and erode campaign performance. Thus, Anomaly ML not only enables marketers to act on real-time insights but also protects the integrity of their data, ensuring that marketing dollars are spent efficiently with a maximized return on investment (ROI).
Enhancing Campaign Efficacy with ML-Driven Insights
Impact of Anomaly ML on User Engagement Metrics
In the digital marketing realm, user engagement metrics are pivotal indicators of campaign success — from click-through rates to conversion ratios. The introduction of Anomaly Machine Learning (ML) in this aspect isn't just innovative; it's revolutionizing. Anomaly ML serves as a meticulous auditor, rigorously validating user interactions to ensure they originate from genuine sources.
- Detection of Bots and Fake Interactions: By analyzing patterns, Anomaly ML can differentiate between behavior indicative of real users and that of bots or scripts designed to mimic human engagement.
- Accuracy in Conversion Rates: Particularly, Anomaly ML sharpens the accuracy of conversion rates by filtering out noise from fraudulent activities, thereby providing a more authentic look at campaign performance.
- Elevated Engagement Signals: Genuine interactions are amplified, ensuring that strategic decisions are based on data that truly represents customer interest and behavior.
Through Anomaly ML, advertising campaigns have seen notable improvements in engagement metrics, which can be attested through various case studies. For instance, a retail e-commerce platform utilized Anomaly ML to identify and discard bot traffic, which resulted in a substantial uplift in the accuracy of their click-through data, ultimately leading to a more effective allocation of their ad budget and resources.
From Numbers to Strategy - Data-Driven Decision Making
With Anomaly ML, not only is the data cleaned from potential fraud, but it also becomes a gold mine for actionable marketing insights. Marketing teams can now, more than ever, rely on their datasets to be reflective of customer behaviors and trends without the noise created by fraudulent activities.
- Enhanced Targeting Precision: By understanding the segments of genuine users, brands can tailor their messaging and segmentation strategies to suit the exact needs of their true audience.
- Optimization of Campaigns: Clean data enables marketers to fine-tune their campaigns in real-time, reducing wasteful spend on non-performing ads and reallocating budget towards high-performing segments.
- Strategic Messaging: When data integrity is assured, messaging can become more personalized and strategic, resonating with the real audience and driving better campaign outcomes.
Painting a picture with clean, anomaly-free data, digital marketing professionals can craft strategies that hit the mark with precision. With Anomaly ML, marketing becomes not just an art but a keen science, pinpointing where and how to engage potential customers most effectively, boosting campaign impact and ROI.
Get started with Verisoul for free
Tackling the Challenge - Integration and Operationalization
Seamless Integration of Anomaly ML in Current Marketing Ecosystems
For digital marketing professionals and AdTech companies, the seamless integration of Anomaly ML into existing marketing platforms is crucial for maintaining operational continuity while enhancing fraud detection capabilities. Often, the inclusion of sophisticated solutions like Anomaly ML can raise concerns about workflow disruptions and increased complexity. However, through best practices in systems integration, such as API connectivity and modular deployment, Anomaly ML can be embedded into the core of marketing ecosystems without compromising user experience or system performance.
- Start by conducting an audit of your current marketing technology stack and assess compatibility with Anomaly ML technologies.
- Utilize APIs to facilitate smooth data interchange between Anomaly ML tools and existing marketing databases.
- Opt for Anomaly ML solutions that offer customizable modules, allowing for a gradual rollout and minimal interference with ongoing campaigns.
Maintaining a user-friendly experience is another top priority, especially for MarTech leaders and Product Managers in the SaaS space. While bolstering security against ad fraud is non-negotiable, it must not come at the cost of usability. The deployment of Anomaly ML should be transparent to end-users, running silently in the background, preserving the fluidity and intuitiveness of user interfaces that customers are accustomed to.
- Ensure that user interfaces remain clean and intuitive, with Anomaly ML functions integrated seamlessly behind the scenes.
- where possible, leverage Anomaly ML insights to enhance user experience through personalized content, avoiding any sense of intrusiveness.
- Provide comprehensive support and training to both team members and clients to aid them in adapting to any new features or procedures.
Refining Detection - The Journey from False Positives to Precision
One of the most significant challenges in the operationalization of Anomaly ML is managing and reducing false positives. False positives, wherein legitimate user actions are flagged as fraudulent, can lead to wasted ad spend and can undermine the trust of customers and partners. Therefore, precision in detection is paramount.
- Start with a robust data cleansing process to ensure that Anomaly ML models are trained on high-quality data.
- Continually retrain and update Anomaly ML models with new data to maintain accuracy and relevance in the face of evolving ad fraud tactics.
- Implement feedback mechanisms where manual reviews can inform the Anomaly ML system, reducing false positives over time.
For Data Science and AI Experts in Marketing, fine-tuning Anomaly ML models is an ongoing process that requires vigilance and responsiveness. Strategies for continually improving model accuracy must be in place, adapting to new data and fraud tactics as they emerge.
- Engage in continuous monitoring and evaluation of the model's performance metrics, adjusting thresholds and parameters as needed.
- Encourage cross-functional collaboration between data scientists, marketing professionals, and cybersecurity experts to refine detection capabilities.
- Foster a culture of innovation within the team, encouraging the exploration of new algorithms and methodologies that can enhance precision.
Remember, the goal is to create Anomaly ML models that not only detect fraud at a high rate but also instill confidence in the legitimate transactions they approve. This balance between security and operational efficiency is the key to maximizing ad spend ROI in an industry rife with sophisticated fraud.
Balancing Fraud Prevention and Marketing Dynamics
The Evolving Landscape of Digital Ad Fraud
In the dynamic battlefield of digital advertising, fraudsters continually adapt their techniques to circumvent traditional detection methods. As a result, the war against ad fraud requires vigilance and ongoing strategy adjustment. Machine learning (ML) models are at the forefront of this struggle, with the capability to analyze data in real time and spot unusual patterns that may signify fraudulent behavior.
Anomaly detection ML, in particular, offers hope in the fight against ad fraud by identifying irregularities within massive datasets that human analysts might miss. A proactive approach, vigilant to the constantly evolving methods of deception, is vital. For instance, advertisement stacking or pixel stuffing are tactics that have managed to infiltrate even the most safeguarded systems; a robust ML model can rapidly spot such deceptions through its learning mechanisms, which evolve to reflect the ever-changing tactics.
The key for these models is to keep pace with the shifting fraud patterns. Historically, as soon as marketers and technologists decode the blueprint of a particular fraud modality, a new scheme materializes. Therefore, dynamic anomaly ML models must learn from incoming data, adapting and improving their detection algorithms to stay ahead of the curve, thus safeguarding marketing investments.
Averting Brand Damage through Proactive AI Security
One of the unspoken casualties of ad fraud is brand reputation. The connection between fraudulent activities and the marketing efforts of a brand, whether direct or indirect, can stain a company’s image if associated with deceitful behavior. Hence, security measures leveraging AI and Anomaly ML are invaluable in preserving the integrity of branding exercises.
The perpetration of fraud doesn't just deplete financial resources but can also mislead marketers about their audience, leading to inaccurate targeting and poorly tailored content. Anomaly ML assists in creating a double-edged sword to not only impede fraudsters but also to facilitate accurate and dynamic marketing strategies. Its ability to discern authenticity in user engagement equips marketers to proactively counteract the potential brand damage before it can take root.
A balanced approach includes a comprehensive AI security strategy that aligns with the brand’s growth ambitions without compromising user experience. Marketers must walk the fine line between stringent detection measures and the natural, frictionless customer journey. By utilizing Anomaly ML's high-power computational proficiency and delicate touch, brands can secure their ad spend ROI while fostering positive and authentic user engagement.
Moreover, active vigilance through Anomaly ML supports the detection of sophisticated schemes such as ad injection and domain spoofing, which, if left unchecked, can severely impair the perceived reliability of a brand. As we push the envelope on AI security in digital marketing, it is becoming clear that a harmony exists between aggressive fraud prevention tactics and the natural flow of effective, dynamic marketing. It's this tailored equilibrium that will dictate the success in leveraging ad spend for genuine growth, negating the noise and interference of fraudulent entities.
Final Thoughts and Next Steps
Maximizing ad spend ROI is a complex challenge that demands innovative solutions, and Anomaly ML has proven itself as a powerful tool in the digital marketing arsenal. As we've explored, this technology not only enhances the integrity of data but also fortifies marketing campaigns against increasingly sophisticated ad fraud schemes.
-
Reevaluate Your Fraud Detection Systems: It's time for digital marketing professionals, AdTech companies, and MarTech leaders to closely assess their current fraud detection strategies. Where are the gaps? How well are you keeping up with the latest in fraudster tactics?
-
Adopt Anomaly ML Technologies: The adoption of Anomaly ML should be seen as a strategic investment—its integration can significantly impact the bottom line by ensuring that ad spend is not siphoned off by fraudsters.
-
Leverage Long-term ML Benefits: Beyond immediate ROI improvements, machine learning offers long-term benefits in terms of data quality, campaign effectiveness, and strategic decision-making. Fraud prevention is not just about protecting current investments; it's about securing future growth.
-
Stay Ahead with Continuous Learning: To remain competitive, it is essential to stay informed about the latest developments in cybersecurity and fraud detection technologies. Engage with cybersecurity experts, attend industry conferences, and participate in professional forums.
Call to Action: For growth hackers, SaaS product managers, and data science experts, now is the time to deepen your expertise in Anomaly ML applications and explore new ways to shield your operations from fraudulent activities. The path to optimized ad spend and solid ROI growth is continuous vigilance and technological adaptation. Take the step today—investigate how Anomaly ML can become part of your organization's cybersecurity framework and help keep your ad spend working for you, not against you.