How Bot Farms Affect Digital Marketers and Advertisers: Crucial Insights
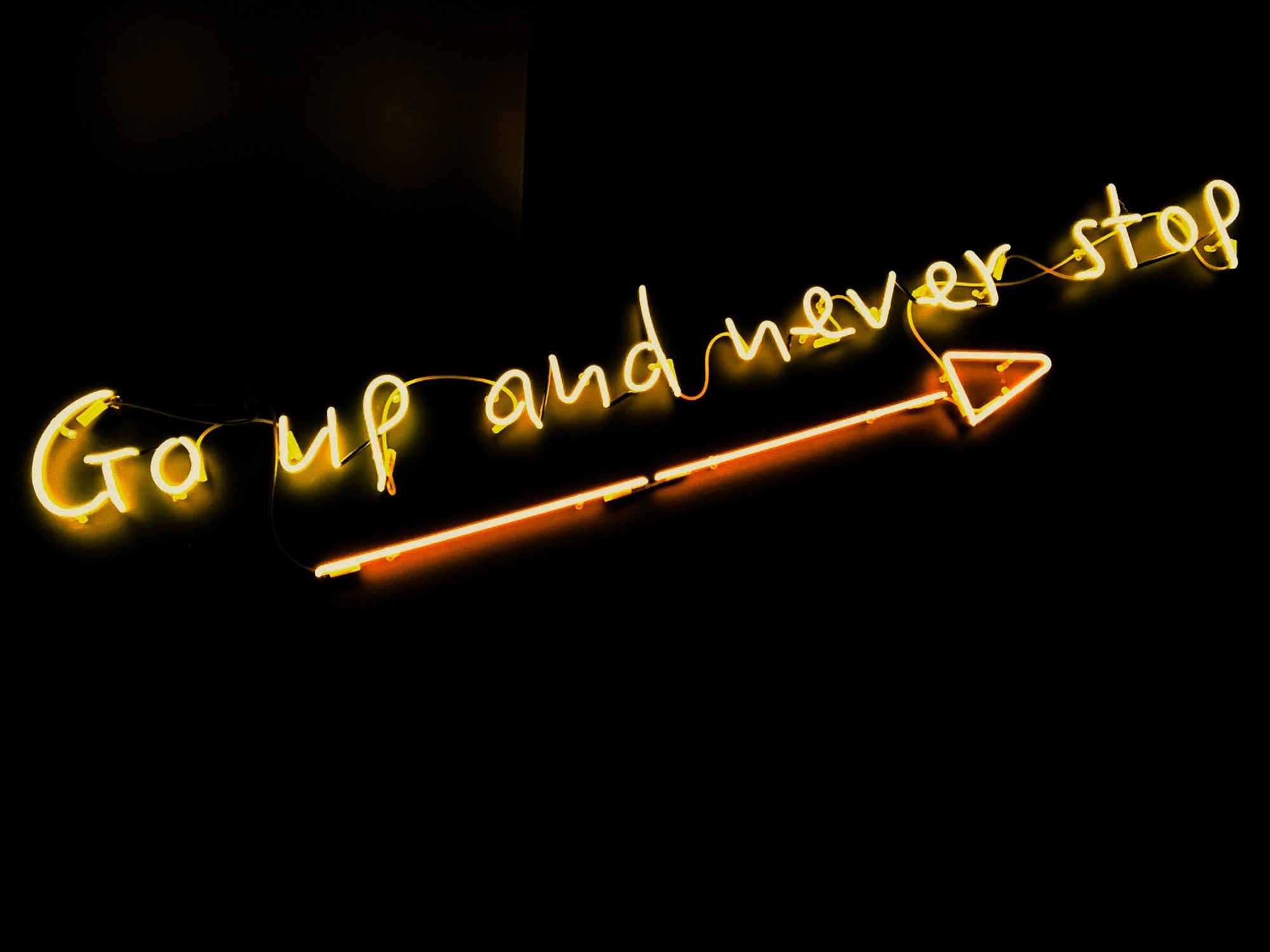
Bot farms have become an increasingly prevalent issue in the digital marketing and advertising landscape, threatening the integrity of these industries and leading to wasted budget and flawed analytics. As networks of fake user accounts, bot farms are controlled by automated software that mimics human behavior, leading to invalid traffic and fraudulent engagements. Their impact on advertising and marketing campaigns can prove to be detrimental to the performance and success of campaigns, affecting the bottom line of businesses and undermining the credibility of ad metrics.
To ensure a legitimate online presence and combat ad fraud, digital marketing professionals, advertisers, brands, webmasters, and ad exchange platforms should stay updated on the latest trends and tactics in identifying and mitigating bot farms' effects. Fraud prevention specialists and cybersecurity professionals should also be well-versed in this area, as bot farms play a significant role in ad fraud, data breaches, and other malicious activities. Verisoul's platform, designed to help businesses, applications, and communities stop fake users, is a valuable resource in addressing these issues.
In this article, we will explore five technical strategies for protecting advertising and marketing campaigns from the influence of bot farms. These strategies will provide the necessary tools and insights for our target audience to effectively safeguard their digital campaigns and platforms from the consequences of bot farms and ad fraud. By delving into the specific aspects of each strategy, our audience can better understand the techniques, pros and cons, and tactical implementation of these methods in mitigating the impact of bot farms on their advertising and marketing efforts. As a result, businesses and organizations can strengthen their defenses and reduce the chance of falling victim to the damaging effects of bot-generated traffic and fraudulent engagements.
Strategy 1: Device and Browser Fingerprinting
What is Device and Browser Fingerprinting
Device and browser fingerprinting is a technique used to identify and track users based on their unique device and browser configurations. This process assigns a unique identifier to every device, which can then be used to differentiate between genuine and suspicious user activities in advertising and marketing efforts.
How it works
- Analyzing device configurations: This includes examining information such as screen resolution, operating system, browser version, and other hardware and software components.
- Collecting browser data: Browser data, including cookies, installed plugins, and user agent, are gathered for analysis.
- Evaluating JavaScript settings: This involves looking into JavaScript configuration settings like timezone, language preferences, and fonts, among others.
- Creating unique identifiers: This step assigns a distinct identifier to each device, based on the analyzed data.
Pros & Cons
- Better ad targeting: By identifying and understanding the users, you can create more targeted advertising campaigns, driving improved results.
- Filtering out fraudulent traffic: Identifying malicious browsers and devices helps filter out fake users, which can improve your ad spend efficiency.
- Ensuring integrity of engagement metrics: By eliminating bot-generated traffic, the integrity of your engagement metrics, such as clicks and conversions, is maintained.
- Potential concerns with user privacy: Some users may view fingerprinting as invasive to their privacy, and certain regulations may restrict its use. It's essential to stay informed and comply with relevant data protection laws.
Tactical Implementation
- Integrating fingerprinting SDKs or libraries: Various SDKs and libraries are available for implementing device and browser fingerprinting, such as FingerprintJS, Augur SDK or OWASP Amass.
- Defining parameters to assess device uniqueness: Decide which parameters to track and capture to create accurate device profiles. For instance, you might analyze the device's user agent string, screen size, and resolution.
- Developing algorithms to analyze collected data: To maximize the efficacy of device fingerprinting, develop custom algorithms or leverage pre-built solutions to analyze collected data and identify unique identifiers.
By understanding the ins and outs of device and browser fingerprinting, digital marketers can mitigate the effects of bot farms, improve ad targeting, and maintain the integrity of campaign metrics. Be cautious about privacy concerns, stay compliant with data protection laws, and fine-tune your implementation to achieve the desired results.
Strategy 2: IP Analysis
What is IP Analysis
IP Analysis refers to the process of examining Internet Protocol (IP) addresses that originate traffic, to detect fraudulent activities and block bot farms. By identifying suspicious IP addresses or ranges, digital marketers and advertisers can more effectively prevent click fraud, domain spoofing, and fake traffic from bot farms.
How it works
IP Analysis involves the following processes:
- Detecting proxy IPs, VPNs (Virtual Private Networks), and datacenter IPs, which are commonly used by bot farms to conceal their location and appear as legitimate traffic.
- Analyzing IP ranges and connections for anomalies, such as a large number of requests coming from a single IP or a small range of IPs. This can indicate the presence of bot farms.
Pros & Cons
IP Analysis offers several advantages and drawbacks for digital marketers and advertisers:
Pros:
- Preventing click fraud and domain spoofing: By identifying and blocking malicious IPs, marketers can ensure that their ad clicks and impressions are legitimate, thereby improving campaign performance and ROI.
- Focusing ad budget on legitimate users: By filtering out fraudulent traffic, marketers can ensure that their ads are displayed to real users, maximizing their marketing budget's effectiveness.
Cons:
- May inadvertently block legitimate users using VPNs: Users with genuine intentions might use VPNs for privacy or security reasons. Blocking VPNs could inadvertently block these legitimate users, reducing overall ad reach.
Tactical Implementation
To implement IP Analysis in your digital marketing and advertising efforts, follow these steps:
- Incorporating IP intelligence APIs: Integrate IP intelligence Application Programming Interfaces (APIs) to assess the origin and, in some cases, identify potential risks associated with IP addresses. Leading IP intelligence providers include MaxMind, IPQualityScore, and Neustar.
- Developing blacklists and whitelists for IP ranges: Create and maintain lists of IP addresses or ranges known to be associated with bot farms (blacklists) and those considered safe (whitelists). This helps filter out unwanted traffic while minimizing the risk of blocking legitimate users.
- Continuously updating IP lists and monitoring traffic for anomalies: Regularly update your blacklists and whitelists with new IPs associated with bot farms, and monitor your traffic for anomalous behavior indicative of bot activity. This will ensure the ongoing effectiveness of your IP Analysis strategy.
Get started with Verisoul for free
Strategy 3: Emulator and Virtual Machine Detection
What is Emulator and Virtual Machine Detection?
Emulator and Virtual Machine (VM) Detection is a technique used by digital marketers, advertisers, webmasters, and security professionals to identify and block fraudulent traffic originating from emulated devices or virtual machines. These devices and machines are often used by bot farms to mimic human actions and create a facade of genuine user engagement, thereby skewing ad metrics, eating up marketing budgets, and compromising overall campaign success.
How it works
Emulator and VM Detection work by analyzing an array of system properties and characteristics that are specific to emulated environments or virtual machines. Some common markers include non-standard device model names, inconsistent memory configurations, lack of hardware acceleration, and absence of certain features that are typically present in physical devices. By comparing these properties against known indicators of emulators and virtual machines, the detection system can identify and block instances that attempt to engage with ads or websites.
Pros & Cons
Pros:
- Identifying and mitigating fake traffic and engagement: Detecting emulators and virtual machines enable advertisers and marketers to filter out fraudulent activities by identifying the devices used by bot farms.
- Protecting campaigns from virtual machine-generated bot traffic: By blocking devices running on emulators and virtual machines, marketers can ensure that only genuine users view and engage with their ads, safeguarding marketing budgets and maintaining the integrity of ad metrics.
Cons:
- May cause false positives in specific cases: Although emulator and virtual machine detection is highly effective, there might be instances where certain genuine users are mistakenly flagged as fraudulent, leading to accidental blocking of legitimate traffic.
Tactical Implementation
To implement emulator and virtual machine detection in your advertising and marketing campaigns, consider the following steps:
-
Utilize detection tools or APIs: Leverage specialized tools, SDKs, or APIs available in the market that detect the use of emulators or virtual machines. These solutions usually enable easy integration with existing systems or platforms.
-
Monitor behavioral patterns and system characteristics: Continuously analyze user interactions, device properties (e.g., screen resolution, CPU/GPU information), and system configurations on your platform to identify common markers of emulators and virtual machines.
-
Regularly update detection algorithms: As new types of emulators and virtual machines emerge, it is crucial to stay abreast of the latest developments and update detection algorithms periodically. This can help maintain high detection accuracy and ensure that your campaigns remain protected against evolving bot farm threats.
By implementing emulator and virtual machine detection, digital marketers, advertisers, and other relevant professionals can more effectively combat bot farms and their negative impact on advertising and marketing efforts.
Strategy 4: Advanced Captcha and Automation Framework Detection
What is Advanced Captcha and Automation Framework Detection
Advanced Captcha and automation framework detection is a technique that helps advertisers and marketers identify and filter out malicious bot traffic by implementing sophisticated challenges for user verification and detecting the presence of automation tools used to manipulate website interactions.
How it works
- Implementing complex challenges for Captcha: To differentiate between human users and bots, advanced Captcha solutions require users to solve more complex challenges before accessing specific website features, such as submitting a form or interacting with an ad. These challenges could involve puzzles, image recognition tasks, and multi-step problems.
- Detecting presence of automation frameworks: Some bot farms use automation frameworks, like Selenium or Puppeteer, to simulate human-like interactions with websites. By identifying server-side requests originating from these frameworks or monitoring for patterns indicative of automation, websites can block suspicious traffic.
Pros & Cons
- Distinguishing between human users and bots: As advanced Captcha challenges are more difficult for bots to solve, implementing these techniques can effectively mitigate bot traffic, safeguarding ad engagements and maintaining campaign integrity.
- Protecting ad engagements: By blocking bot interactions, advanced Captcha and automation framework detection effectively reduce the risk of click fraud and wasted ad budgets.
- Enhanced website analytics: With fewer bot interactions, website owners and advertisers gain a more accurate understanding of user engagement and performance metrics, enabling better data-driven decision-making.
- May add friction to user experience: The complexity of advanced Captcha challenges might increase the difficulty for legitimate human users, potentially leading to user frustration and abandonment of website tasks.
Tactical Implementation
- Integrating advanced Captcha solutions: Implement solutions such as Google's reCAPTCHA, hCaptcha, or FunCaptcha into your website or advertising platform, ensuring the Captcha challenges are sufficiently complex to deter bot interactions.
- Monitoring server-side requests for automation patterns: Analyze server-side requests for signatures or patterns that may indicate the presence of automation frameworks or bot traffic, such as specific user agent strings or headers.
- Fine-tuning Captcha challenges to balance security and user experience: Continuously monitor the impact of Captcha challenges on real users and bot traffic, making adjustments as necessary to maintain a balance between security and user satisfaction.
By adopting advanced Captcha and automation framework detection techniques, digital marketers and advertisers can effectively combat the negative impacts of bot farms on their campaigns. This strategy requires a meticulous approach to implementation and continuous improvement, ensuring that websites can effectively filter out malicious bots without compromising the experience for legitimate users.
Strategy 5: Behavioral Analysis and Bot Behavior Biometrics AI
What is Behavioral Analysis and Bot Behavior Biometrics AI
Behavioral analysis and bot behavior biometrics AI refer to the process of leveraging artificial intelligence (AI) and machine learning (ML) algorithms to analyze and differentiate human and bot patterns based on user interactions and engagements. This approach assesses the nuances of user behavior, including response times, keystroke patterns, scrolling speeds, and other interactions, to identify suspicious or questionable behavior associated with bot farms.
How it works
Behavioral analysis and bot behavior biometrics AI techniques work by collecting vast amounts of data on user interactions and behavior, such as mouse movements, clicks, swipe gestures, and typing patterns. AI and ML algorithms then analyze this data to create biometric profiles of legitimate human users. By comparing new user interactions against these profiles, the algorithms can determine if the user is genuine or an automated bot or bot farm.
Pros & Cons
Pros:
-
Reducing ad fraud: By effectively differentiating between human and bot behavior, marketers and advertisers can reduce fraudulent traffic generated by bot farms and better allocate their resources towards genuine users.
-
Improving overall marketing ROI: With plugging the leaks caused by bot farm-engaged ad fraud, businesses can witness an improvement in their returns on marketing investments, ensuring that their advertising dollars go further in reaching their target audience and generating conversions.
Cons:
- Potential for misidentification: As bots and bot farms evolve and adapt their behavior to convincingly mimic humans, there is a risk that the AI algorithms may misidentify genuine users for bots or vice versa. A robust implementation must account for these dynamics to minimize errors.
Tactical Implementation
To implement behavioral analysis and bot behavior biometrics AI effectively, digital marketing professionals should pursue the following steps:
-
Implement AI-driven analytics tools: Choose and integrate an AI or ML-driven analytics tool that can process large volumes of user interaction data and create biometric profiles for detecting bots and bot farms.
-
Monitor user interactions for behavior patterns: Continuously monitor user interactions through your chosen analytics tool, collecting as much data as possible on variables such as user interaction speed, click patterns, and more. This information will allow the AI algorithms to discern discrete bot behavior signatures.
-
Refine AI models with constant feedback and learning: To stay ahead of the evolving tactics of bot farm operators, it is essential to provide continuous feedback to the AI models to improve their accuracy and effectiveness. Close collaboration between end-users and the providers of AI-driven analytics tools is necessary to ensure timely updates and refinements to the ML algorithms.
By implementing this strategy, digital marketing professionals will be better equipped to combat the impact of bot farms on their advertising and marketing efforts. As the landscape of ad fraud evolves, staying vigilant and adaptive to advancements in AI and ML-driven behavior analysis is crucial for long-term success.
Final Thoughts and Next Steps
In conclusion, bot farms pose a significant threat to digital marketers, advertisers, and platforms by generating fraudulent traffic, fake engagement, and manipulating ad revenues. To combat this, we have discussed five technical strategies:
- Device and Browser Fingerprinting - Analyzing device configurations and browser data to create unique identifiers
- IP Analysis - Detecting suspicious IP addresses and monitoring traffic for anomalies
- Emulator and Virtual Machine Detection - Identifying and blocking instances of virtual machines and emulators generating bot traffic
- Advanced Captcha and Automation Framework Detection - Implementing complex Captcha challenges and detecting automation frameworks
- Behavioral Analysis and Bot Behavior Biometrics AI - Leveraging AI to analyze user actions and differentiate between human and bot behavior
It is crucial for industry professionals to adapt to the evolving landscape of ad fraud and stay updated on the latest trends and techniques in combating bot farms. This involves continuously monitoring and improving their anti-fraud tactics and investing in new technologies and solutions.
Moreover, a comprehensive and layered approach to ad fraud prevention is recommended. By combining multiple strategies to protect against different types of bot farm attacks, businesses can more effectively safeguard their advertising budgets, marketing campaigns, and user experiences. Implementing this information can help mitigate the impact of bot farms and create a safer and more secure digital ecosystem.