Government IT Leaders Harness Anomaly ML for Fraud Prevention
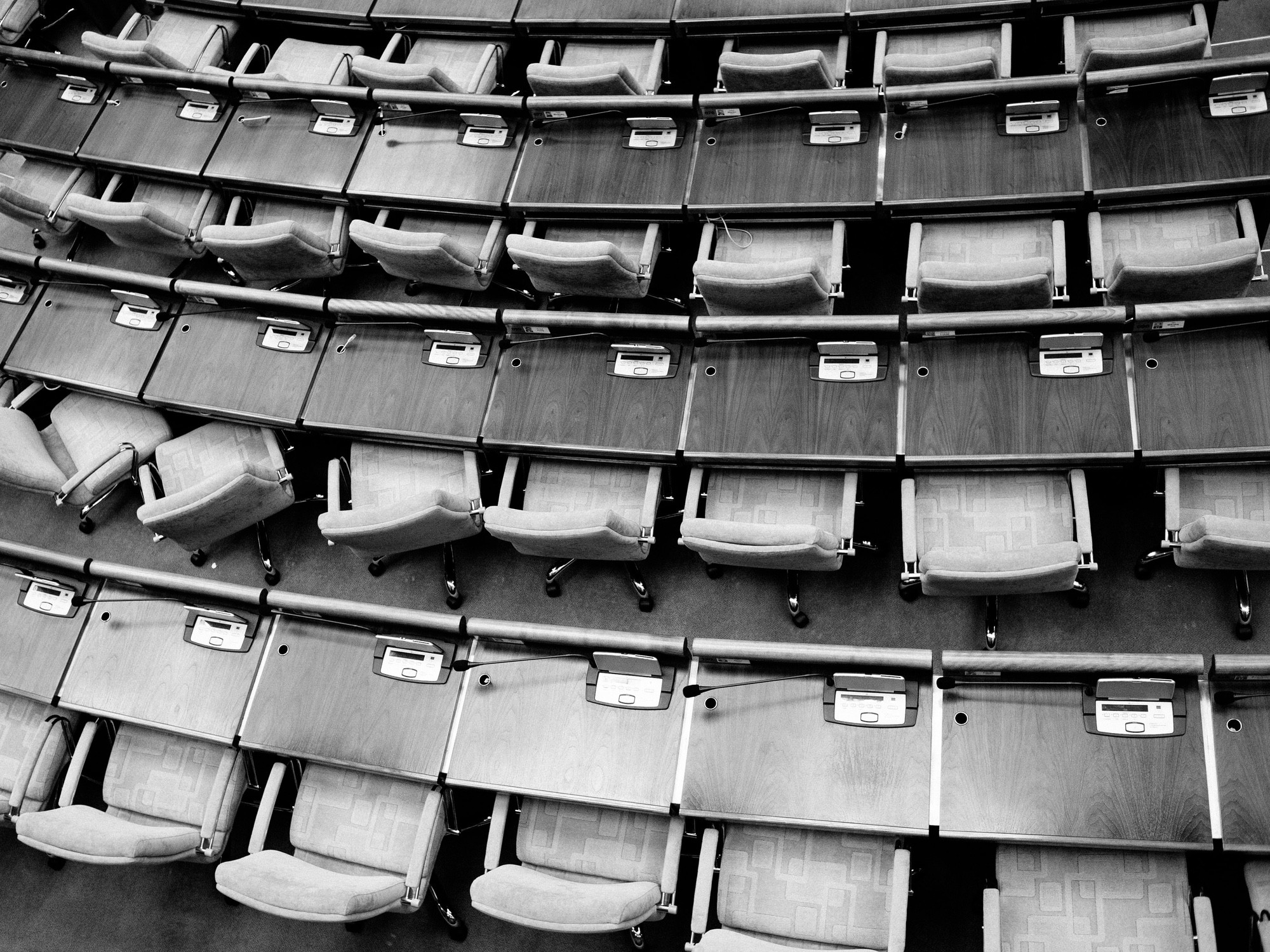
Reliable fraud prevention measures are the backbone of maintaining public trust in government services. As fraud tactics evolve, so must the tools and methodologies used to detect and prevent illegal activities. Anomaly Machine Learning (ML) has recently stepped into the spotlight, offering a formidable arsenal against the scourge of identity fraud and fake users that plague the public sector.
Government IT leaders are at the vanguard of this technological battle. By integrating Anomaly ML into their security systems, they set a new standard for proactive fraud prevention. This approach is not just about keeping pace with digital adversaries. It's about staying several steps ahead, leveraging advanced algorithms that can sift through vast volumes of data, recognising patterns invisible to the human eye.
The implications are profound. Enhanced security protocols mean a reduced burden on resources, freeing up funds and manpower for other critical areas of public service. For cybersecurity professionals, this translates to a layer of defense that is continuously learning and adapting—an invaluable asset for agencies besieged by sophisticated cyber threats. Meanwhile, fraud detection officers may find in Anomaly ML an ally that never tires, sifting tirelessly through transactions for the faintest hint of fraud.
As digital transformation advocates watch closely, this technological pivot is not just about preventing fraudulent activities. It is as much about underlining an ever-renewable commitment to the protection of citizen data. In the hands of program and policy managers, Anomaly ML could signal the dawn of a new era in government transparency and efficiency—an era where innovation is the cornerstone of trust and security.
With these considerations in mind, understanding the role of Anomaly ML in fraud detection becomes imperative for these public sector leaders. This article aims to provide that comprehension in a clear and detailed manner.
Fundamentals of Anomaly ML in Fraud Detection
Definition of Anomaly Detection and Machine Learning
Anomaly Detection is a critical process within the cybersecurity and fraud prevention toolbox of public sector organizations. It involves identifying patterns in data that do not conform to expected behavior, which are labeled as anomalies. These can signal potential instances of fraud or security breaches, making anomaly detection a valuable ally in maintaining the integrity of government systems.
Machine Learning (ML), a subset of artificial intelligence, is applied to anomaly detection to discern normal from abnormal patterns reliably. There are three primary types of ML used: Supervised Learning, where models learn from labeled data to recognize fraud; Unsupervised Learning, which detects anomalies without pre-labeled data; and Semi-Supervised Learning, that uses a combination of labeled and unlabeled data to improve detection accuracy.
Data Acquisition and Model Training
For Anomaly ML to function effectively, acquiring high-quality, labeled datasets is paramount. These datasets form the foundation upon which ML models are trained, and the quality and relevance of data directly influence the model's performance. Government agencies must ensure that data is comprehensive and representative of the real-world scenarios where fraud might occur.
The training of ML models for anomaly detection is a meticulous process where data is fed into algorithms to help them 'learn' from it. Over time, these models can identify subtle patterns and correlations that human analysts might overlook. As they are continuously refined through exposure to new data, their accuracy in detecting fraudulent activities is enhanced.
Real-Time Analysis and Decision-Making
Once an ML model is trained, it is capable of analyzing new transactions and user activities in real time. This is crucial for the quick identification of potential fraud, allowing government agencies to take prompt action before significant harm is done. These models help in handling the vast volume of data and transactions typically seen in the public sector.
Algorithms play a pivotal role in rapid and accurate decision-making. They are designed to process vast amounts of information and provide insights that might indicate fraudulent behavior, thus enabling fraud detection and prevention efforts to be both efficient and effective. Advanced algorithms coupled with machine learning enable government agencies to stay one step ahead of fraudsters, providing a proactive defense mechanism in the ongoing battle against fraud.
Benefits of Implementing Anomaly ML for Government Fraud Prevention
Enhanced Detection Capabilities
One of the critical advantages of integrating Anomaly ML in the public sector is the enhanced detection capabilities it offers. Machine learning models are adept at adapting to new and evolving fraud tactics over time. As fraudulent schemes become more sophisticated, ML models can continuously learn from the data they process, allowing for the detection of nuances in behavior that might signal fraudulent activity. This evolution in detection is essential in staying ahead of malicious actors who are constantly updating their methods to bypass traditional checks.
Moreover, Anomaly ML is inherently scalable, capable of handling the vast datasets that are typical of government operations. As service users grow in number, it is imperative to have a system that can keep up with the volume of data, and ML models are well-suited to scale with those demands. Here are some specific scalability benefits:
- ML algorithms can process millions of transactions in real-time.
- They have the capacity to identify patterns across disparate data sources.
- Scalability allows for cross-agency data sharing and analysis, leading to a more comprehensive detection net.
Reduction in Operational Costs
The automation of fraud detection processes is another significant benefit. By deploying Anomaly ML, government agencies can reallocate resources typically involved in manual reviews and investigations. This not only speeds up the fraud detection process but also reduces the operational costs associated with personnel hours and potential overpay in fraudulent claims. Automating routine tasks further allows staff to focus on more complex and investigative matters that require human expertise.
Ensuring Citizen Trust and Privacy Compliance
In an era where data privacy is of utmost concern, utilizing machine learning for fraud detection also supports the public sector's commitment to citizen trust and privacy compliance. Anomaly ML models are enhanced with privacy-preserving techniques that allow them to learn from data without compromising the individual's personal information. This aligns with regulatory standards, such as the General Data Protection Regulation (GDPR), and reinforces citizens' trust in public services.
Ensuring the security of personal data must be a priority for any fraud detection system, and ML allows for just that. This technology not only safeguards this information but does it in a way that doesn't impede the ability to effectively identify and prevent fraud. Here are a few privacy-preserving techniques often used in ML:
- Data anonymization and encryption to prevent unauthorized data identification.
- Differential privacy, which adds random noise to datasets to mask individual contributions.
- Secure multi-party computation, which enables entities to jointly analyze data while keeping the inputs confidential.
By adopting Anomaly ML, government entities endorse a proactive stance against fraud and show dedication to maintaining the integrity and security of their systems, all while upholding the privacy and trust of their citizens.
Get started with Verisoul for free
Challenges and Mitigation Strategies
Model Interpretation and Transparency
For government IT leaders and cybersecurity professionals in the public sector, the push towards using Anomaly ML for fraud prevention is often met with challenges surrounding model interpretation and transparency. Explainability in ML models is critical, especially for auditability in public sector applications where stakeholders require a clear understanding of how decisions are made.
Across the board, from fraud detection to compliance officers to program and policy managers, the demand for transparency can be high. The use of complex algorithms, which may act as "black boxes", can make it difficult to trace how a model arrived at a particular conclusion. This can complicate compliance with regulations and increase the risk of mistrust among citizens.
To improve model transparency for auditing purposes, several tools and practices can be employed:
- Implementation of simplified models that can be easily interpreted by non-experts.
- Adoption of techniques that provide insights into model predictions, such as LIME (Local Interpretable Model-Agnostic Explanations) or SHAP (SHapley Additive exPlanations).
- Ensuring that ML outputs can be translated into comprehensible reports for regulatory bodies and other stakeholders.
These strategies aim to demystify ML decision-making processes, thus fostering accountability and maintaining public trust.
Balancing Privacy Regulations and Data Utilization
The interoperability between anomaly ML applications and privacy regulations is a growing concern for those in the public sector. With laws like the General Data Protection Regulation (GDPR) and California Consumer Privacy Act (CCPA) imposing stringent requirements for data handling and user consent, ensuring that ML-based fraud detection systems adhere to these laws is paramount.
Techniques such as federated learning can address privacy concerns by allowing ML models to be trained across multiple decentralized devices or servers holding local data samples without exchanging them. This approach is particularly useful for public sector entities that handle sensitive personal data across different jurisdictions.
To effectively implement anomaly ML, the public sector must:
- Develop policies that balance the need for data utilization against the individual's right to privacy.
- Structure ML systems to be capable of operating within the confines of existing privacy regulations.
- Invest in privacy-preserving technologies to maintain the integrity of ML solutions.
Resource Constraints and Training Requirements
Anomaly ML systems are data and resource-intensive. Government entities might struggle with the infrastructure costs and the human capital required to effectively use ML for fraud detection. Upgrading the technological capacity for implementing and maintaining ML solutions can be a significant challenge, especially under tight budget constraints.
Furthermore, there is a pressing need to upskill the existing workforce to adapt to new ML technologies. Digital transformation advocates and IT leaders face the task of planning for continued education and professional development to cultivate the necessary expertise within their teams.
Strategies for addressing resource and training challenges include:
- Earmarking funds specifically for the adoption of ML technologies to mitigate fraud.
- Seeking partnerships with academic institutions and private-sector entities for knowledge transfer and resource-sharing.
- Organizing targeted training programs and workshops to enhance the ML competency of staff.
Through these mitigation strategies, the public sector can overcome several of the challenges related to the adoption of anomaly ML for fraud detection, ensuring that investments into ML are cost-effective, respectful of citizens' privacy, and lead to improved operational integrity.
Future of Anomaly ML in the Public Sector Context
Trends in Machine Learning and Predictive Analytics
The landscape of machine learning (ML) and predictive analytics in the public sector continues to evolve rapidly, shaped by advances in Artificial Intelligence (AI) and Big Data technologies. Government IT leaders and cybersecurity professionals are harnessing sophisticated algorithms capable of processing vast amounts of data to detect anomalies and potential fraud. These systems can adapt to emerging fraud tactics over time, learning from new data patterns to improve the accuracy of predictions.
- Integration with AI: Combining ML with AI to enhance decision-making processes.
- Use of Big Data: Leveraging large, diverse datasets for more nuanced and reliable anomaly detection.
As technology progresses, anomaly ML systems become more adept at highlighting unusual patterns that deviate from established norms, ensuring that public sector entities remain vigilant against fraud in an increasingly digital environment.
Collaborative Efforts Across Agencies for Integrated Fraud Detection
For anomaly ML to be truly effective in combating fraud, it must transcend organizational barriers. Data silos within government agencies can impede the ability to detect sophisticated fraud schemes that span across different systems and jurisdictions. To remedy this, there is a growing push for collaborative efforts across agencies.
- Information Sharing: Initiatives aimed at secure sharing of data between entities.
- Cross-Agency Platforms: Developing integrated platforms that enable holistic fraud detection.
By pursuing partnerships and collective approaches, public sector agencies can form a more formidable defense against fraudsters, ensuring that anomaly ML tools have access to comprehensive data to identify and counteract fraudulent activities efficiently.
Perspective on Innovation and Procurement in Government
In the world of government IT, aligning the pursuit of technological innovation with the mandates of fiscal responsibility presents a unique challenge. IT executives must reconcile the need for cutting-edge anomaly ML solutions with prudent budget management. In light of this, public sector agencies are becoming more strategic in their procurement processes, seeking cost-effective solutions that do not compromise on performance.
- Value-driven Procurement: Emphasizing the long-term ROI of ML technologies in fraud prevention.
- Flexible Funding Models: Exploring subscription services, as-a-service offerings, and public-private partnerships.
The policy and program managers focused on innovation must navigate this complex landscape, ensuring that investments in ML contribute meaningfully to the primary goal of fraud prevention. By maintaining a balance between innovative technology adoption and fiscal caution, government departments can continue driving forward in their fight against fraud using anomaly ML tools.
Final Thoughts and Next Steps
In the journey towards equipping government systems with the most effective defenses against fraud, Anomaly Machine Learning (ML) does not just stand as an option; it is quickly becoming an indispensable tool. We've circled around the substantial benefits and challenges that this technology presents to the public sector, balancing fraud prevention capabilities with operational cost reductions and compliance needs.
Continuous Improvement and ML Advancements
- Government IT leaders must not rest on current laurels but advance with the pace of ML innovation.
- Regularly update anomaly detection algorithms to counter the ever-evolving fraud techniques.
- Allocate budgets for ongoing training and data quality enhancement to strengthen model accuracy.
Encouraging Inter-Agency Collaboration
- Bolstering efforts to prevent fraud through Anomaly ML calls for a collective approach.
- Sharing insights, data, and strategies can lead to a more robust and unified defense against fraudulent activities.
- Establish cross-agency task forces that specialize in ML-based fraud detection strategies.
Educating and Involving Stakeholders
- Invest in a broad cybersecurity awareness program to educate staff and citizens about their role in fraud prevention.
- Public sector leaders should look to involve every layer of their organization—from frontline workers to top executives—in understanding and supporting ML solutions.
- Regular workshops and forums can help disseminate vital knowledge and best practices across departments.
In conclusion, the stride towards a fraud-resistant public sector is continuous and requires a steadfast commitment to technological advancement, collaboration, and education. Government IT leaders stand at the helm of this transformation, steering their agencies towards a future where Anomaly ML not only identifies fraud but propels the integrity and trust of public services to unprecedented heights.