DeepFake Detection for Community Platforms Thwarts User Fraud
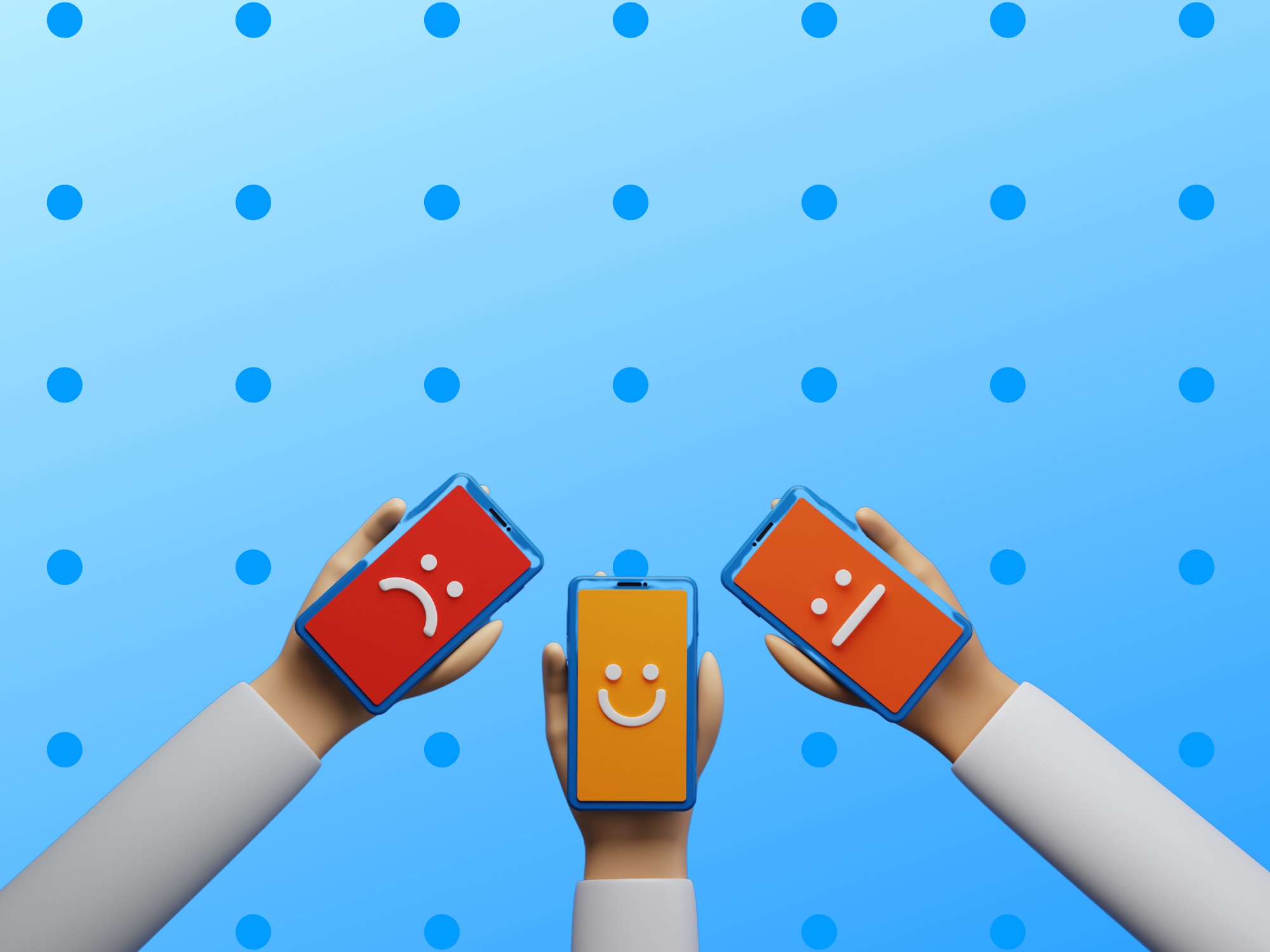
Digital communities serve as thriving hubs for interaction, commerce, and innovation, becoming core to product-led growth in contemporary businesses. As these platforms gain prominence, they unwittingly attract nefarious activities, including synthetic identity fraud. The sophistication of these fraudulent schemes has advanced with the advent of deepfakes, which use artificial intelligence to create hyper-realistic yet entirely fictional representations of humans.
These challenges necessitate a robust solution to maintain trust and security within digital ecosystems. Deepfake detection technology has emerged as this pivotal solution. Not only does it act as a deterrent to fraudsters, but it is also crucial for community platforms committed to authentic connections.
For decision-makers and technical professionals, understanding the operational benefits of integrating deepfake detection is essential. With the integrity of user interactions at stake, deploying such technology is not only a measure against current threats but a foundational step towards a future-proofed platform. The aim is to ensure each member of the digital community is genuine, their contributions are authentic, and the shared space remains a stronghold against synthetic disruptions.
By aligning with deepfake detection solutions, tech-savvy leaders, security experts and community managers can reinforce their commitment to creating secure, trustworthy environments where innovations and interactions flourish without the looming threat of cyber deception.
The Growing Threat of Deepfakes in Digital Communities
Understanding Deepfakes
Deepfakes are hyper-realistic digital manipulations of video or audio, typically used for creating convincing fake content that portrays real people saying or doing things they never did. Cybercriminals use advanced artificial intelligence (AI) and machine learning to generate deepfakes, making them a significant threat in online communities where trust and authenticity are paramount. In these digital spaces, users expect interactions to be genuine, but deepfakes can severely undermine this trust.
The creation of deepfakes involves using generative adversarial networks (GANs), where two AI models work in tandem—one to create the fake images or videos and the other to detect flaws. This process repeats until the generated media is indiscernible from authentic content. As deepfakes become increasingly sophisticated, detecting them with the naked eye becomes nearly impossible. For cybercriminals, deepfakes represent a powerful tool for identity theft, the spread of misinformation, and the commission of fraud within communities built around user-generated content.
The Impact on Community Platforms
The repercussions of deepfake technology within online communities can be extensive and multifaceted:
-
Data Security: Personal data can be exploited to create deepfakes, leading to potential breaches of sensitive information. This compromises the security measures community platforms have in place to protect user data.
-
User Experience: The presence of deepfakes on a platform can lead to a toxic environment, driving away genuine users and tarnishing the community's reputation, which is pivotal for user retention and growth.
-
Legal Compliance: There is an increased risk of legal action against platforms that fail to address the dissemination of deepfakes, which can be construed as negligence in protecting users from digital harms.
-
Community Engagement: Deepfakes can cause mistrust among users, erode the credibility of shared content, and disrupt the organic exchange of ideas and engagement that are the hallmarks of thriving online communities.
-
Loss of Trust: Once users’ trust is compromised, it can lead to a downward spiral of engagement and value, potentially resulting in a loss of revenue and user base.
Without effective strategies to combat deepfakes, community platforms risk damaging their brand, losing the trust of their user base, and facing legal challenges. Therefore, deepfake detection is not merely an option—it's an essential component of safeguarding online ecosystems from fraudulent activity that threatens to dissolve the very fabric of digital trust.
Unpacking Deepfake Detection Technology
Detection Mechanisms
In the ongoing battle against deepfakes in community platforms, detection mechanisms serve as the vanguard. AI methodologies are at the core of this technological frontier, employing advanced algorithms to discern genuine from synthetic media. A critical component in this arsenal is facial recognition technology, which analyzes the nuances of human facial movements and contrasts them with the often too-smooth articulations of AI-generated faces. The fine details often overlooked by the human eye, such as unnatural blinking patterns or incoherent lip-syncing, become red flags for AI detectors.
Another layer of defense is behavioral analysis. This technique goes beyond the facial structure, looking for inconsistencies in the way individuals speak or move. Subtle cues like the natural head tilt in conversation or the rhythm of someone's breathing can be telltale signs of a deepfake, as AI has yet to perfectly mimic the complexities of human behavior.
Lastly, GAN (Generative Adversarial Network) fingerprinting has become an influential tool. Since many deepfakes are created with GANs, these networks leave behind unique digital fingerprints. Detection systems can be trained to identify these fingerprints, and as such, can reveal whether a video or image has been manipulated. This form of detection is continually being refined as GAN technology evolves, making it vital for cybersecurity and fraud prevention strategies.
Integration and Scalability
For community platforms, seamless integration of deepfake detection into existing tech stacks is essential. Solutions must be versatile enough to work with various types of media content and user interaction models. Many deepfake detection platforms offer APIs that easily connect with the community's existing infrastructure, causing minimal disruption to service and allowing for rapid deployment.
However, it is the scalability of these solutions that is particularly crucial. As platforms grow, the volume of user-generated content inevitably increases. It is here that scalable deepfake detection technologies exhibit their true value. They are designed to handle an expanding data volume without a drop-off in performance. This implies that the speed and accuracy of the detection mechanisms are maintained—or even improved—as the workload grows.
Modern deepfake detection solutions utilize cloud-based infrastructures to ensure that as the number of users swells, the system's capability to process and analyze media in real-time does not wane. This is paramount because as platforms gain popularity, they become larger targets for fraudulent activities. Having a system that can grow with the user base while maintaining strict security standards is indispensable for tech-savvy leaders seeking to safeguard their communities.
In summary, whether it's through the precision of facial recognition, the intricacies of behavioral analysis, or the identification of GAN fingerprints, the state-of-the-art in deepfake detection stands as a bulwark against the erosion of trust within digital communities. Coupled with the capabilities for smooth integration and scalability, these technologies represent a significant investment in the future security and integrity of online platforms.
Get started with Verisoul for free
Advantages for Community Integrity
The deployment of deepfake detection in community platforms can significantly enhance the authenticity of user interactions and protect the integrity of the digital interaction space. By accurately differentiating between real human contributions and those fabricated by AI technologies, platforms ensure that their users engage in a safe environment that fosters trust and genuine human connection.
Key Advantages:
- Enhanced Security Posture: Implementing deepfake detection mechanisms arms platforms against a sophisticated breed of identity fraud, thereby strengthening the overall defense against cyber threats.
- Compliance with Regulations: As global governance tightens around digital identity verification, such as GDPR and the California Consumer Privacy Act (CCPA), integrating deepfake detection tools aligns community platforms with legal requirements, mitigating risks of non-compliance.
- Maintenance of User Trust: Ensuring that interactions within a community are legitimate upholds user trust, which is paramount in a digital landscape where reputation can significantly impact user retention and growth.
Navigating the Hurdles
While the benefits are substantial, there are several considerations that platforms must address when implementing deepfake detection technologies. Key among these is the complexity of the systems involved.
Computational Resource Requirements:
- Larger platforms may require significant processing power to analyze high volumes of interaction in real time.
- As deepfake technology evolves, detection tools must be continually updated, demanding additional resources.
User Experience Considerations:
- It's vital to maintain a delicate balance between tight security measures and fluid user experience to prevent user frustration.
- False positives or negatives can lead to wrongful user suspension or permitting fraudulent actors, respectively.
Striking a Balance:
- Implementing a threshold for detection sensitivity can help in reducing inconvenience to legitimate users.
- Incorporating a robust appeal and support process ensures that users feel supported even when detection mechanisms inadvertently flag genuine content.
By thoughtfully addressing these hurdles, community platforms can integrate deepfake detection without impairing user experience or operational efficiency, creating a resilient ecosystem that values both security and user satisfaction.
Strategic Implementation within Business Operations
Aligning with Business Goals
Incorporating deepfake detection technology into the framework of a community platform transcends mere adoption of a security feature; it is a strategic maneuver aligned with overarching business goals. For tech-savvy leaders and product-led growth advocates, this means anchoring the technology firmly within the context of enhancing user trust, ensuring robust community engagement, and underpinning marketable features that distinguish a platform as advanced and secure.
- Strengthen User Trust: By integrating deepfake detection, platforms signal their commitment to authentic interactions, bolstering user confidence.
- Enhance Market Position: Employing this technology can position a community platform as a leader in security innovation, appealing to security-conscious professionals.
- Optimize Regulatory Alignment: With compliance managers ever-watchful of changing regulations, deepfake detection aids in remaining compliant with digital identity verification laws.
Deepfake detection technology can act as a catalyst for growth, with community managers experiencing firsthand the reduction in fraudulent activities, leading to cleaner, more engaged user interactions. The tangible improvements in community quality can then be leveraged as a unique selling proposition in product development and marketing strategies.
Best Practices for Integration
Successful implementation of deepfake detection dovetails with best practices in software integration and operations management. Below are actionable recommendations that community platform architects and cybersecurity experts can apply to ensure a seamless integration that enhances platform integrity without disrupting the user experience.
- Iterative Implementation: Roll out the technology in phases, starting with high-risk areas, to manage system load and optimize detection algorithms.
- Invest in Computational Efficiency: Equip your infrastructure to handle the intense computational requirements of real-time deepfake analysis, ensuring robust scalability.
- User-Centric Design: Create user interfaces and interactions that inform but do not alienate users, making security checks a seamless part of their experience.
- Continuous Learning Framework: Integrate the detection technology with a feedback loop to allow for continuous learning from new data, reducing false positives and adapting to new deepfake techniques.
- System Optimization: Regularly review and refine deepfake detection capabilities to ensure they are up-to-date with the latest threats, maintaining a robust security posture.
Above all, engage with your community users by soliciting their feedback on security measures. This input is invaluable in striking the right balance between stringent security and user convenience, as community managers and moderators become the frontline defense in ensuring the authenticity of users and content.
Through strategic implementation, business operations can harness deepfake detection not only as a defensive mechanism but also as a pathway to drive user engagement, establish market differentiation, and achieve product-led growth.
Final Thoughts and Next Steps
In the age of rapidly evolving cyber threats, the integrity of digital communities hinges on the ability to authentically verify each user. The prevalence of deepfakes presents a clear and present danger to this integrity, which deepfake detection technology effectively mitigates.
Emphasizing User Authenticity:
- Trust is Paramount: Ensure every interaction on your platform is authentic to uphold user trust.
- Security is Essential: Proactive measures against deepfakes are critical for robust cybersecurity postures.
Advancing with Deepfake Detection:
- Combat Fraud: Employ deepfake detection to safeguard your community from synthetic identity fraud.
- Preserve Community Values: Maintain the core principle that each member is real, unique, and engaged in genuine interaction.
Strategically Embracing Technology:
- Integrate Seamlessly: Choose solutions that integrate smoothly with your existing tech stack and user experience.
- Stay Scalable: Opt for technologies that grow alongside your platform, accommodating expanding user bases and data volumes.
Continuous Vigilance and Adaptation:
- Monitor Threat Landscape: Stay informed about the evolving nature of cyber threats and prepare to counteract them.
- Upgrade and Optimize: Regularly update your fraud detection systems to combat the most recent and sophisticated threats.
In conclusion, the adoption of deepfake detection technology is not just a tactical maneuver but a strategic imperative for community platforms aiming to foster a secure, authentic, and trustworthy environment. Community platform stakeholders should remain agile, embracing detection technologies and maintaining vigilance in their cybersecurity efforts. By doing so, platforms can not only thwart user fraud but also establish themselves as pioneers in the fight against digital impersonation. The next step is clear: evaluate and integrate a powerful deepfake detection system to safeguard the future of your platform and its community.