Datacenter Detection Essentials for Secure Community Platforms
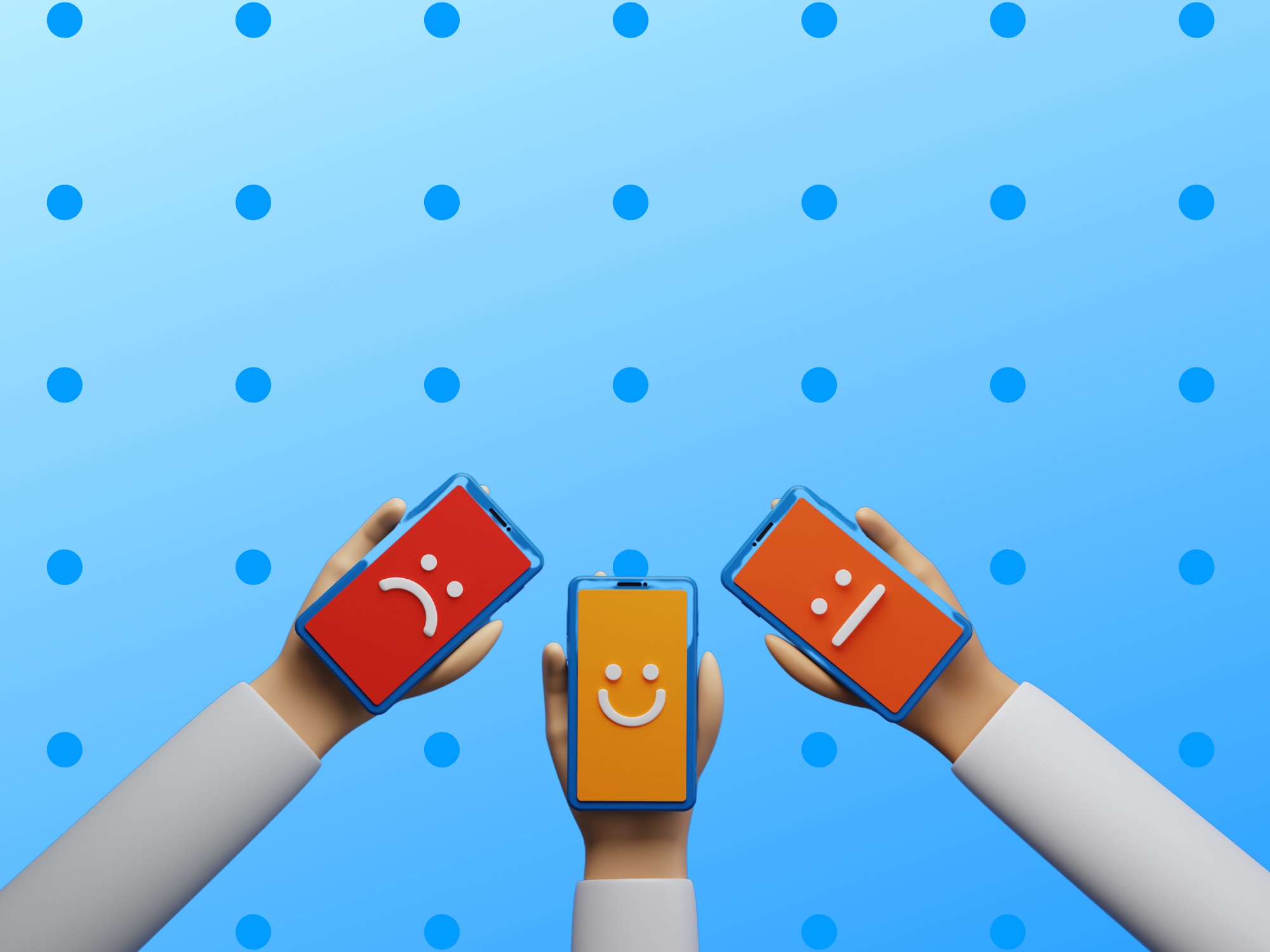
The integrity of online community platforms is vital to the growth and user retention of tech-savvy companies. Product managers and community architects constantly seek robust defenses against fraudulent activity. Datacenter detection has emerged as a powerful tool in maintaining user authenticity and safeguarding digital communities. As businesses grow, so do the challenges associated with keeping community interactions genuine. The presence of bots, compromised accounts, and other malicious entities can quickly erode trust and compromise the user experience.
Technical leads and developers find themselves at the forefront of integrating datacenter detection into their security strategies. The technology's ability not only to spot but also to preemptively block high-risk IP addresses is fundamental in the fight against online fraud. As community managers endeavor to cultivate high-quality user engagement, the importance of real-time threat detection becomes all the more evident.
For IT security professionals, the approach to digital security within the company must be proactive and multifaceted. Datacenter detection provides a critical layer of security that complements other defense mechanisms. These professionals need scalable, efficient, and, most importantly, effective tools. With datacenter detection as part of the technological arsenal, community platforms stand a better chance at thwarting the efforts of fraudsters and maintaining the kind of secure environment that's imperative for a thriving online community.
The Landscape of Online Community Fraud
The Threat: Types of Fraudulent Activities
In the intricately connected realm of online communities, various forms of fraudulent activities pose serious challenges to platform integrity and user trust. Bots are scripted programs that simulate human activity, often flooding platforms with spam or skewing engagement metrics. Fake accounts, created en masse, can distort user demographics and manipulate content popularity or visibility, while Sybil attacks involve the creation of multiple identities to subvert reputation systems or to carry out coordinated disinformation campaigns.
It's paramount to recognize that such activities have serious repercussions. They go beyond mere nuisances, leading to broader implications like eroded user trust and compromised data integrity. For product managers and IT security professionals, this can result in decreased user engagement, tarnished brand reputation, and potential leaks of sensitive user information.
Technical Sophistication of Modern Fraud Techniques
Fraudsters today employ highly sophisticated techniques to sidestep traditional detection methods. Polymorphic bots, for instance, can alter their identities to evade signature-based detection tools, while the pervasive use of VPNs enables malicious users to obscure their origin, complicating efforts to filter traffic based on geographic profile.
Furthermore, impersonation and behavioral mimicry represent advanced threats where malicious entities emulate legitimate user behavior. Here, identifying nefarious intentions becomes particularly challenging, as these entities can blend in without raising immediate suspicion. This technical sophistication demands equally advanced defense strategies, prompting a need for nuanced detection mechanisms capable of discerning legitimate users from masquerading actors.
The fight against community fraud is relentless, with perpetrators continuously refining their methodologies. For developers and community managers, this means employing proactive and dynamic solutions that evolve at pace with, or ideally ahead of, the latest fraudulent techniques. Datacenter detection stands out as a critical tool that, when correctly integrated and maintained, can significantly mitigate the risks associated with these sophisticated forms of online fraud.
Datacenter Detection: The First Line of Defense
Defining Datacenter Detection
In the context of online communities, datacenter detection plays an essential role in distinguishing between genuine user activity and automated traffic that can be nefarious in nature. It functions as an automated traffic filtration and bot mitigation system aimed at identifying and blocking behaviors originating from data centers, which is a common source of illegitimate activities such as bot-driven fraud. Datacenter detection is especially critical in protecting the integrity of user interactions and maintaining a secure environment for community platforms.
Anatomy of a Datacenter Detection System
Understanding the components of an effective datacenter detection system is crucial. At its core, IP analysis is the bedrock upon which this technology stands, scrutinizing the origin of each request to determine if it's from a datacenter. Traffic pattern monitoring is equally important, as it watches for unnatural sequences of actions that might indicate automated processes rather than human users.
Behavioral analysis goes hand-in-hand with machine learning algorithms to create a nuanced security measure that can adapt over time. This two-pronged approach allows for a more dynamic response to evolving threats while reducing the risk of false positives that can hamper the experience of legitimate users.
- IP Analysis and Traffic Pattern Monitoring: Key for identifying potential non-residential IP traffic and recurring patterns synonymous with bots.
- Behavioral Analysis: Utilizes machine learning to understand user behavior nuances and identify discrepancies pointing to automated sources.
- Machine Learning Algorithms: Evolve to recognize new threats and adapt to sophisticated attacker tactics.
Integration into Community Platforms
For decision-makers, the question of integrating datacenter detection into their platforms is as essential as the tool itself. API-based implementation is a favorable strategy, given its ability to seamlessly integrate into almost any platform without a significant overhaul. This method allows for real-time analysis and decision-making based on traffic type.
Compatibility with current technology stacks is non-negotiable. A datacenter detection service must work alongside existing security measures without causing disruptions to service. Fortunately, most providers design their solutions to be highly adaptable to a wide range of technologies, making integration into existing platforms straightforward:
- API-based Implementation Strategies: Ensures seamless integration with minimal modifications required for existing infrastructure.
- Compatibility with Contemporary Technology Stacks: Guarantees that datacenter detection can be part of a cohesive security ecosystem already in place.
The efficient implementation of datacenter detection helps maintain a secure community platform, contributing to the overall health and sustainability of digital ecosystems powered by authentic user engagement.
Get started with Verisoul for free
Advantages of Implementing Datacenter Detection
Bolstering Security Measures
For tech-savvy companies, the introduction of datacenter detection has revolutionized the way security measures are implemented. With the ability to preemptively block high-risk IP ranges that are commonly associated with datacenters known for malicious activities, platforms can significantly reduce the likelihood of bot-driven fraud. This proactive stance on cybersecurity is crucial since it allows companies to maintain the integrity of their user base and protect against various types of automated attacks that can lead to data breaches or compromised system integrity.
By identifying and filtering traffic originating from suspicious IP addresses, community platforms can thwart the efforts of fraudsters before they inflict damage. This layer of security is especially critical considering that bots and various impersonation techniques continue to evolve, becoming more sophisticated in their evasion tactics.
Enhancing User Experience
A direct benefit of implementing datacenter detection is a notable enhancement in user experience. Genuine users of online community platforms value an environment free from spam, phony engagement, and fraudulent content. Maintaining a high-quality user experience is essential for product-led growth, as it fosters trust and encourages active community participation.
Removal of unwanted bot activity not only secures the platform from potential threats but also ensures that the engagement metrics are reflective of real user interactions. This authenticity is paramount for product managers who rely on accurate data to make informed decisions regarding the direction and evolution of their product features.
Scalability and Performance
The challenge of managing high-volume traffic without compromising on latency or system performance is an ongoing concern for growing platforms. Datacenter detection, with its ability to rapidly analyze and filter traffic, aligns perfectly with the requirement for scalability and performance.
The seamless integration of datacenter detection tools ensures that even during peak traffic times, platforms are capable of distinguishing between genuine user engagement and automated traffic. The tools are designed to handle large data volumes efficiently, thereby minimizing the risk of system overloads or slowdowns that can negatively impact user experience.
By incorporating machine learning algorithms and behavioral analysis into the framework of datacenter detection systems, platforms can adapt to new threats proactively. This continuous learning process not only improves the accuracy of threat detection over time but also ensures that the security measures evolve in tandem with the emerging tactics of fraudsters, without causing disruption to genuine user activity.
In summary, the integration of datacenter detection within community platforms offers a comprehensive approach to preemptively counteract security threats, enhance genuine user engagement, and sustain system performance at scale. This solidifies the platform's reputation as a secure and reliable environment, thereby fostering growth and user retention.
Critical Considerations and Limitations
Balancing Security with User Accessibility
When deploying datacenter detection as part of your cybersecurity strategy, it's crucial to maintain equilibrium between robust security measures and ensuring legitimate users are not inadvertently blocked from accessing your community platform. One common challenge is the management of false positives, which refer to genuine users misidentified as fraudulent by security systems. False positives can arise due to legitimate users accessing the platform through VPNs or shared networks that are often used by fraudsters to cloak their activities.
To effectively navigate this challenge, it's essential to implement a system that can discern patterns indicative of authentic behavior, even when the IP address might raise a red flag. An adaptive authentication process can help in this regard, prompting for additional verification when a user is detected coming from a high-risk IP range but exhibiting legitimate user behavior.
Bullet points to consider:
- Implementing strict security measures without impeding on user accessibility
- Strategies for reducing false positives, such as adaptive authentication
- The importance of accurately distinguishing between legitimate users on VPNs and potential fraudsters
Ongoing Maintenance: Staying Ahead of Fraudsters
Another critical aspect of deploying datacenter detection is the ongoing maintenance required to ensure the system remains effective against evolving fraud techniques. Cybersecurity is not a set-it-and-forget-it affair; it demands continual vigilance. The IP address databases that help identify potential datacenter traffic must be regularly updated to keep pace with the agile nature of fraudsters who frequently change their IP addresses to avoid detection.
Furthermore, the machine learning models that power behavioral analysis must be continuously trained on new datasets to adapt to the ever-changing tactics of sophisticated fraudsters. Machine learning algorithms can become stale if not regularly fine-tuned, leading to a decline in detection accuracy over time.
Bullet points to consider:
- The necessity of updating IP databases to maintain the effectiveness of detection
- Continuous training and refining of machine learning models to counteract evolving fraud techniques
- Establishing protocols for regular reviews and updates of the system's intelligence
Technical Integration Challenges
Integrating datacenter detection into existing systems is not without its technical challenges. The primary concern is ensuring the stability of your community platform while you integrate and configure the new security layer. It's imperative that the implementation of datacenter detection does not compromise the existing user experience or cause downtime, which could negatively impact user retention and trust.
Minimizing implementation complexity is also a key consideration—datacenter detection solutions should align with your current technology stack and be as plug-and-play as possible. An API-based integration approach facilitates this by allowing a more seamless incorporation of cybersecurity measures without the need for extensive modifications to your platform's architecture.
Bullet points to consider:
- Maintaining platform stability and uptime during the integration process
- Choosing solutions that align with the existing technology stack for easier integration
- Leveraging API-based strategies to reduce implementation complexity and integrate swiftly
Understanding these limitations and considerations is essential for a successful implementation of datacenter detection. Addressing them with a tactical approach will help secure your community platform against fraudulent activities without sacrificing user experience, maintainability, or performance.
Final Thoughts and Next Steps
In the digital age where community engagement is critical to product success, datacenter detection stands as a vital component in the arsenal of security tools. It ensures that the user authenticity and interaction within these platforms are protected and genuine—a cornerstone for fostering user trust and sustaining a thriving environment for community-driven growth.
Emphasizing the Importance of Datacenter Detection in Community Management
- It shields your platform from the sophisticated fraud tactics that are ever-evolving.
- Serves as a layer of defense to maintain the quality of user-generated content and interactions.
- Acts as a deterrent against the erosion of user trust, which is fundamental for community longevity.
Continuous Improvement and Security Protocols as a Holistic Approach
For robust security, it is imperative that cybersecurity measures be seen as a continuous journey rather than a one-off setup. Datacenter detection is best utilized within a layered security approach that includes:
- Regular updates to security protocols and filters.
- Continuous training of machine learning algorithms based on new data and trends.
- Real-time monitoring of traffic to identify and address potential threats promptly.
Starting Points for Technical Leads Looking to Implement Datacenter Detection
- Begin by evaluating your platform’s specific needs, assessing user patterns, and identifying the types of fraudulent activities that you are most susceptible to.
- Choose a datacenter detection service that offers seamless API integration, minimal latency, and the capability to handle high-volume traffic.
- Formulate a sound integration plan that includes thorough testing phases to minimize disruptions and ensure stability.
Implementing datacenter detection is a key step in your community platform’s journey to creating a safe and authentic environment. To keep ahead of the curve, remain vigilant, proactive, and agile in adapting your cybersecurity strategies to the changing landscape of online threats. Remember, the safety and integrity of your platform ultimately translate to user retention and product growth.